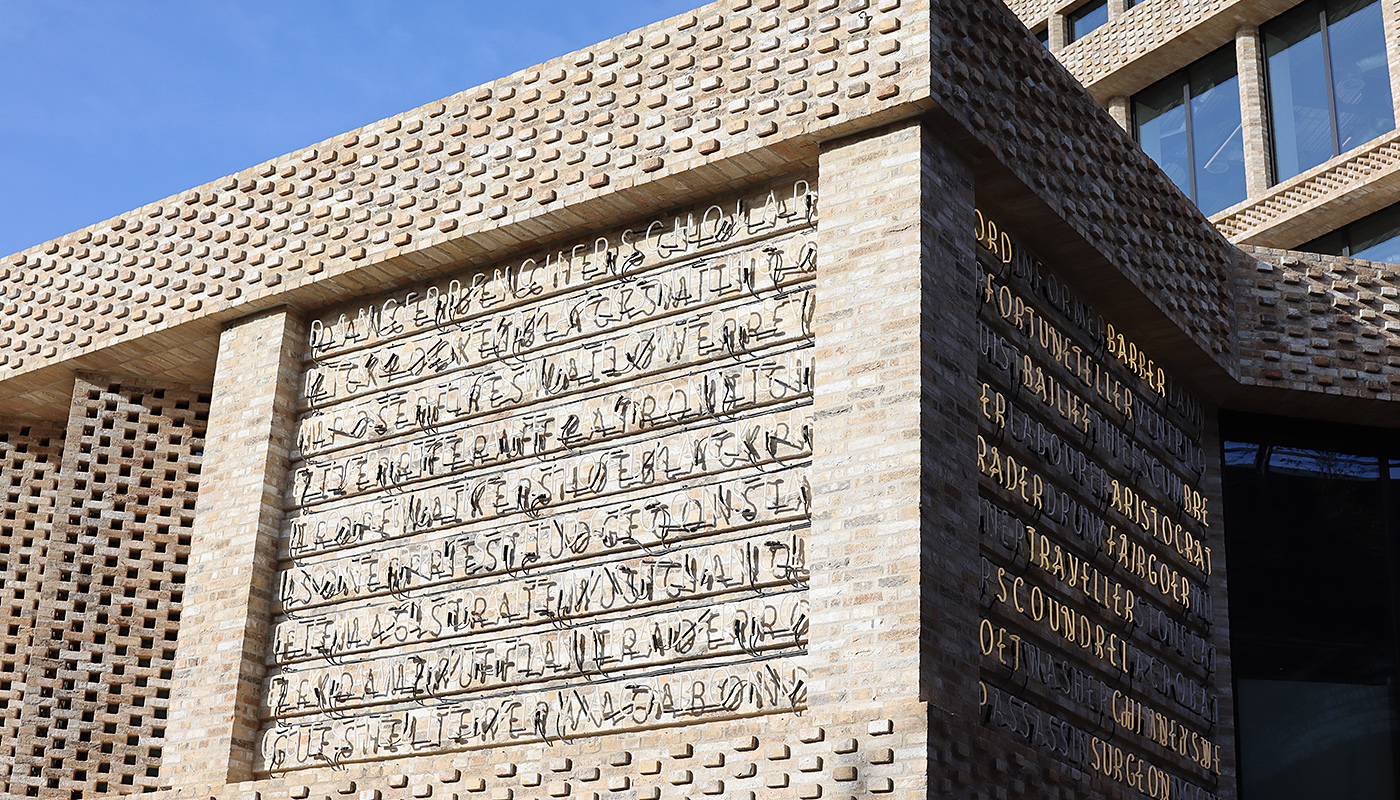
During the pandemic, a question of where to travel to, hasn’t been uppermost in most of our minds. After all, there’s little point in thinking of travelling when it’s been so difficult, and when our minds have been occupied elsewhere, particularly if you’ve been impacted most by the coronavirus, through illness or through work, such as for frontline workers. Slowly, as things have become more “normal” in some parts of the world, travel numbers have picked up, and folks are thinking about holidays again, and asking that question of “where shall I go?”
Obviously, asking questions is something not purely relegated to leisure time. I recently read an article by my friend Firas Marafie on “The Art of Questions” in his new Substack called “Decipher”, essentially about how half the battle is asking the right questions, giving several examples from a business context. It got me thinking more broadly about how we ask questions when it comes to researching financial markets and in particular quant research. It’s definitely something that has spurred our thoughts at Turnleaf Analytics, which Alexander Denev and I recently cofounded to generate macroeconomic forecasts using machine learning and alternative data, such as inflation.
The simpler research questions, more often than not, tend to be more difficult to answer. For example, if an investor asks a question like “how do I make money?”, it’s a pretty straightforward question. However, the possible answers become so broad, it becomes impossible to know where to start. By trying to set parameters around the question it becomes more manageable. How do we want to invest? What asset classes can we trade? Can we trade at high frequencies? Can we trade more illiquid assets etc.?
In particular, if we try to divide up the question into more manageable parts, the problem becomes more tractable, and indeed this idea comes up in many disciplines (eg. divide and conquer in computer science). Let’s say we want to forecast bond yields. We first need to brainstorm what factors impact bond yields. This could range from pure price based variables that measure trend. It is likely to include economic data, in particular inflation and so on.
You might also try to see if you can model these individual factors, or find additional datasets to help, following this idea of breaking down the question into more manageable parts. Of course, you cannot possibly model every single thing, so need to always make a judgement call between building and buying datasets/signals. This will hopefully speed up how quickly you can answer each mini question which arises.
So in the case of inflation as a factor, you could for example contact a vendor, who creates their own inflation forecasts (like Turnleaf Analytics!). The general point though is that you can try to find proxies and in particular more forward looking versions of the same variables, whilst also carefully managing your time. A “perfect model” isn’t really going to be much use, given it takes forever to develop (and probably doesn’t exist anyway!). By comparison a reasonable model which can be researched and developed quickly. It can then be put into production to get insights. Whatever you do, cleaning and understanding your data is key, and you can’t ignore this step, although, using more structured data can help here.
Of course, we also need to bear in mind that many questions we ask, may not have a positive answer. We might spend time doing research, and finding that a particular hypothesis doesn’t fit. However, we should always view this positively, because it has likely to saved you money, knowing to avoid a certain trading strategy. Ideally, of course, we can find a model which performs well historically, but for which we are also confident that will work in the future.