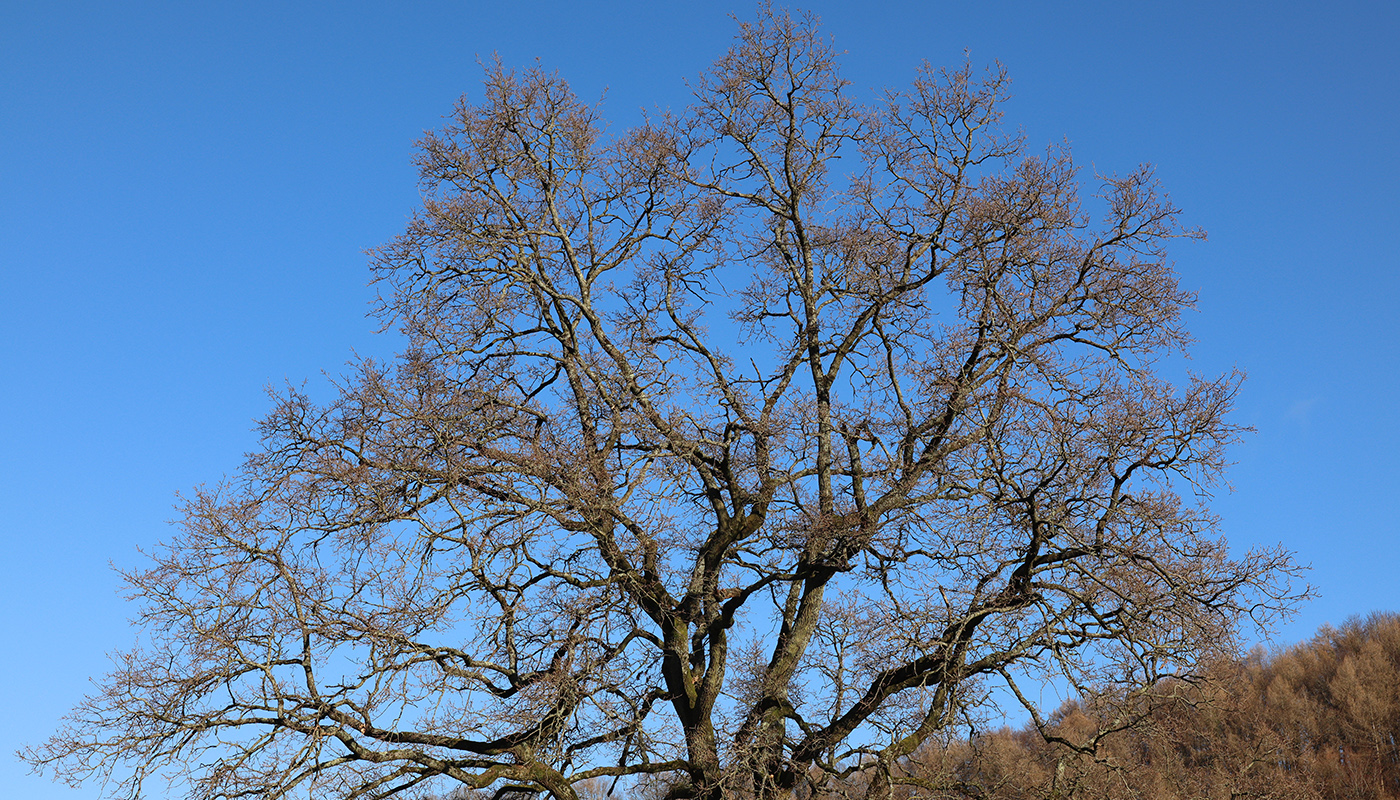
Sometimes, it’s obvious what you want to buy. If you go to a burger joint, you probably want to buy a burger, right? No one goes to a burger joint for a salad (or at least I don’t). However, if we take a step back, what type of burger you’ll order, depends on the place. Go to a Burger King, and it’ll be a Whopper or some variant. If you go to some fancy burger place, there’s going to be more options and the price will be higher too. But let’s say you just want something to eat, and haven’t stipulated we want a burger. Suddenly, the number of restaurants you could visit is going to be much higher and the choices of what you’ll actually order is no longer simply restricted to burgers. You could have sushi, curry etc. pretty much any food available.
But what about when it comes to buying data, how does a buy side (or sell side firm) go about buying data? It’s a question Alexander Denev and I wrote about in The Book of Alternative Data, and it’s something we’ve been thinking about a lot recently, with Turnleaf Analytics, a new firm we co-founded to sell inflation forecast data for emerging markets. Read on, and I’ll explain what burgers have got to do with understanding how much to pay for data if you’re on the buy/sell side? (in the book, we also explain the cost of data from the perspective of a data vendor too)
The burger joint data case – more commoditised data
When it comes to buying data, you have many choices too. The first case (our burger case) is where you know what type of data you need and potentially it might be more commoditised. Let’s say you want to backtest a trading strategy with high frequency data for FX. You’ll be on the look out for high frequency tick market data to purchase. You’ll go through the various providers of this data. This could be the large data firms, such as Bloomberg or Refinitiv, to see what their offerings are. You could also approach ECNs. The datasets will vary in terms of granularity and quality (I won’t go into which ones you should buy, because I haven’t actually looked at a huge number of these datasets!). However, by and large, I would say that they will display quite a lot of similarities. Hence, given the datasets are similar, it is easier to compare the pricing. Essentially, the more commodised a dataset, the easier it is to gauge the cost, because there is a market of many similar datasets out there. Ultimately, with market data, if you don’t have it, you can’t really do any sort of additional analysis too, so it’s not “optional”.
The any restaurant data case – more alternative data
The second case (ie. the non-burger case) of buying data is much more challenging. You might have a hypothesis for a market question you wish to answer. For sake of argument, let’s say we want to forecast Walmart sales. In this instance, unlike the tick data case, there isn’t one specific type of data. There could be many different types of dataset that could be relevant, many of which are alternative datasets (in addition to more standard equity earnings datasets you’ll need). It could be satellite imagery of Walmart car parking lots. It could be machine readable news for Walmart. Or alternatively, we might seek to get location data around Walmart stores, credit card transaction data. Our problem of buying data becomes larger, because we have both multiple vendors and multiple data types. The prices that you could pay for all these datasets are likely to vary quite a bit, given they are so different, despite the fact that are you trying to use them to solve the same question.
The question of how much you should pay for them, will depend upon how much they can improve your forecast or as another external source to crosscheck and compare your own forecasts. We can evaluate the datasets one by one. However, it might be the case, that one specific dataset in isolation doesn’t help, but in combination with others, does provide some notable increase in accuracy, because the dataset is orthogonal to others that you have. Perhaps a better approach is to try several in your Walmart forecasting model, to see how adding an additional dataset can add incremental value. If the “signal-to-cost” ratio is small, then you can dump it. You also need to factor into your cost, the time it takes for researching a dataset, cleaning and structuring it (and of course the ongoing support costs).
Conclusion
In The Book of Alternative Data, we wrote about how to value a dataset in our book, and we provided a handy checklist for data to gauge it’s cost before you do any research work (eg. frequency, what assets it is relevant for etc). It’s obviously difficult to know the exact price you should pay for a dataset. However, if it’s commoditised and there are several vendors who give very similar datasets, it can be easier to gauge a price. It is much more challenging when it comes to alternative datasets, where the nature of the datasets vary. Understanding the incremental value of adding a dataset to your models is crucial. Furthermore, you need to factor into it the cost of doing the research and ongoing support of a dataset. When evaluating datasets, as in our Walmart example, it should be noted, that whilst looking at them one by one can be helpful, we should also understand the incremental value a particular dataset gives our models. There can also be a considerable value in having external forecasts as a means of comparing against ones you have constructed internally (such as those from Turnleaf Analytics!)