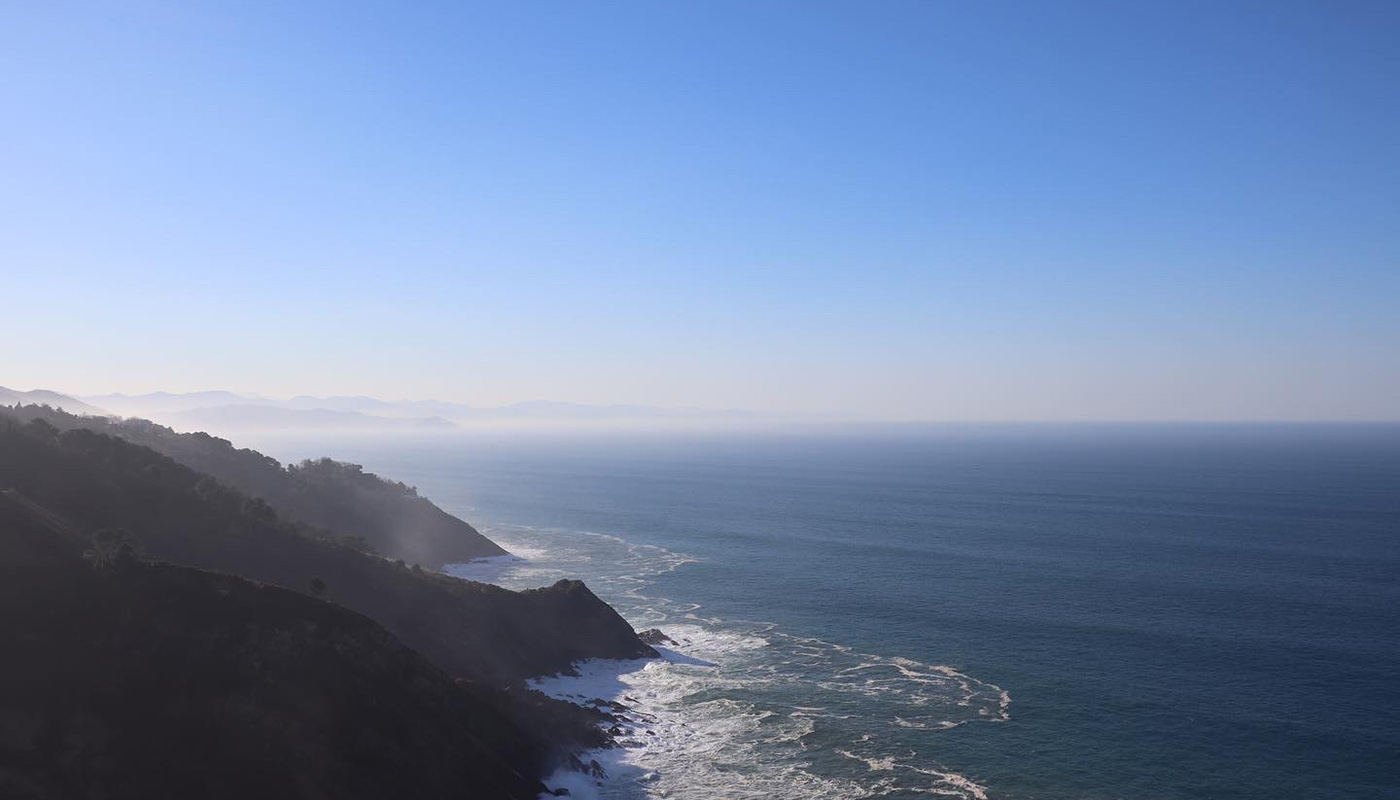
Remembering the past isn’t always easy. I can remember the very worst P&L days of models when I was in a bank (and precisely what triggered the event). Can I remember the best P&L days..? Not a chance. Does that mean there were no good P&L days? Thankfully there were! With burgers, by contrast, I most definitely do remember the very best burgers I’ve eaten, more than the worst. I’m not sure whether I can come to an agreement as to which are my top three burgers though. That’s too difficult!
Over many months Alexander Denev, with whom I cowrote The Book of Alternative Data, and I have been working on forecasting on inflation for emerging markets. It’s for a new company Turnleaf Analytics, which we cofounded. Trying to create forecasting models for inflation (or indeed many other market variables) isn’t easy. However, even before we got to the point of creating a model, we needed to collect and clean the data, which was a very time consuming process. Indeed, depending on the type of model you create, this step of cleaning and collecting the right data can be a massive project in itself. When I say “predicting the past” I basically mean having a dataset which accurately represents what happened on a point-in-time basis, to make sure we can “replay” the past.
Depending on the specific type of dataset, there are different issues with getting access to point-in-time data. I’ll try to go through a few potential issues to be mindful of. The first is that timestamps might be incorrect or lacking detail. If you have daily data, typically they won’t record the precise time of day (depending on your time horizons, “precise” timestamps have different meanings). If you are mixing and matching different data sources, you need to be aware of the fact that “daily” could still mean different times of day.
In FX, you might have London morning snapshots for some Asian currencies, New York close for others etc. The result is that for example, calculations like correlations will appear slightly odd. Some currencies may appear less correlated than practice, because the closing prices are at different snapshots. Other times, the time zone of a timestamp might not necessarily be what you think it would be. For economic data we need to distinguish between the timestamp, which relates to when that economic data was released, versus the period it refers to. Furthermore, we need to take into account that we can have multiple releases for the same number, related to revisions.
One “easy” thing with FX is that the assets don’t really change much. True, we had the introduction of the Euro in 1999, and the occasional inclusion (or exclusion) of a currency into the EM universe, but by and large they are rare events. In equities, we often have IPOs for new companies, and they start trading on stock markets. Conversely, we have companies which stop trading. They could have gone bust, or alternatively gone private. If we use a dataset for equities we need to make sure that we are including all companies that we are trading at a specific point in time. Otherwise, any historic analysis will get skewed, because we’ll be ignoring any companies which went bust (or indeed any which went through an IPO).
It’s not just accuracy of the timestamps themselves, but also of the values associated with that, such as prices. Has the data somehow been rounded excessively before recording? Are they any odd outliers? Are these outliers real data points, or were they some artefact of the recording process?
There is no specific rule for “predicting the past” or ensuring that you have a properly collected point-in-time dataset, which is clean. However, the above represent some potentional things to bear in mind when using historical data. If you dataset is of poor quality, then any analysis you do such as a backtest could end up being unrealistic.