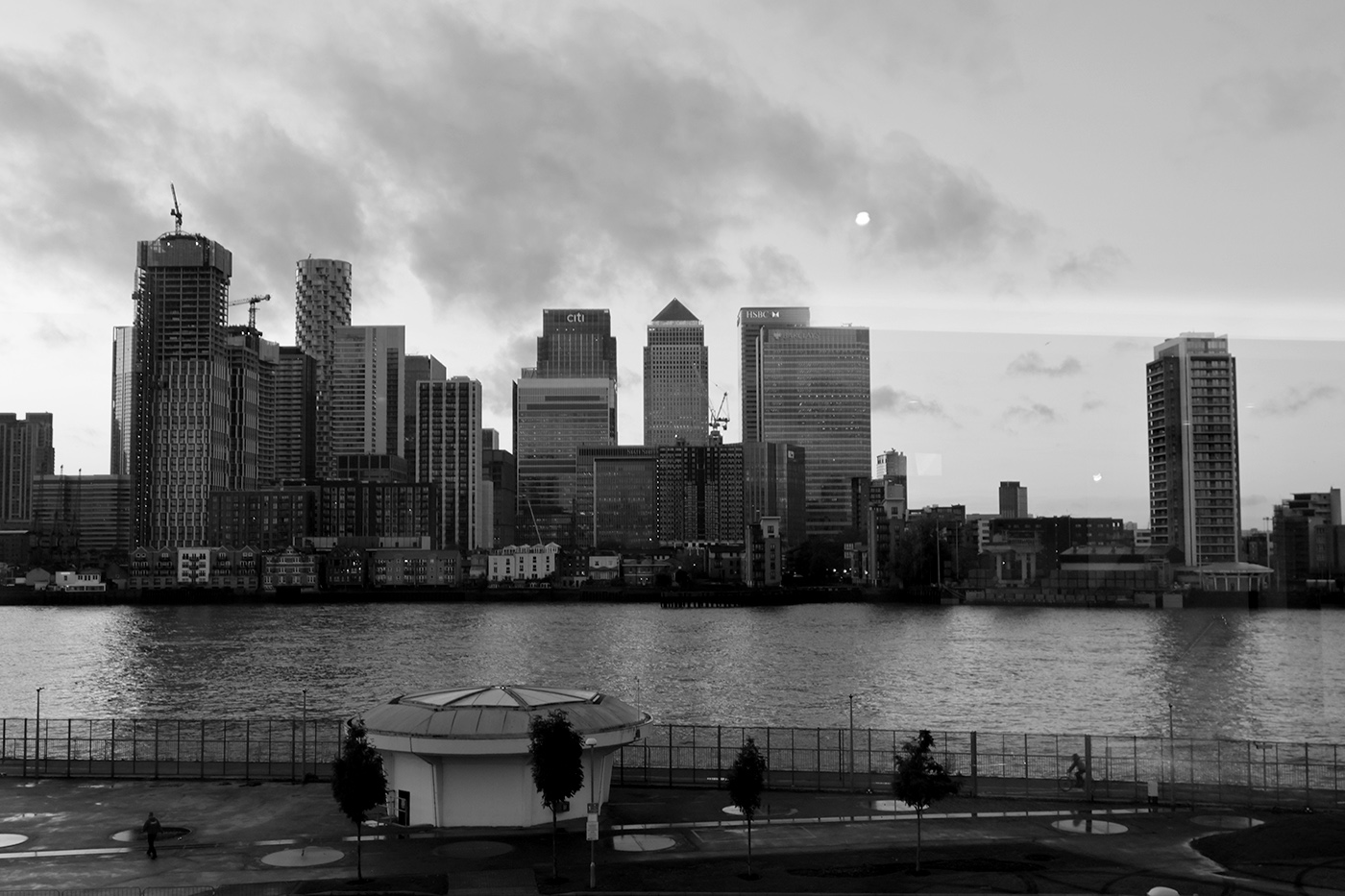
It’s been exactly a decade since I attended my first QuantMinds event. At the time, it was called Global Derivatives, and as the name suggests, it was very much focused towards option pricing and its associated areas. It was held for a number of years in Amsterdam, at the Okura Hotel (whilst I can’t remember what the burgers were like, I can safely say the sushi was excellent year), and then rotated amongst a number of other European destinations ranging including Budapest, Lisbon, Vienna and latterly Barcelona. As the locations changed so did the focus of the conference. The name changed to QuantMinds, in recognition of the fact that the event was covering a wider array of topics, alongside the traditional areas of quantitative finance such as options pricing. Indeed it was notable that John Hull, author of one of the most famous books on option pricing, was teaching a workshop on machine learning at QuantMinds, and has also written a book on machine learning.
This year the event moved to London, and was hosted together with RiskMinds, which is QuantMinds sister event. In terms of the quant calendar, QuantMinds is definitely one of the biggest quant events in terms of attendees but also in terms of the number of speakers at the event, which at times straddles four different streams. As well as the talks, the breaks also allowed for an opportunity to catch up with friends in the industry and to discuss developments.
So what were some of my takeaways from the event and in particular the many presentations at QuantMinds? In this article, I’ll try to articulate a few of them, but sake of brevity, I obviously won’t be able to summarise every single talk. Also furthermore, I’m writing it from the perspective of not being an expert on many of the topics (I doubt anyone can be an expert on all the topics discussed at QuantMinds!). Perhaps unsurprisingly the topics of AI and machine learning came up in a umber of talks, and across many different use cases. Use cases included for LLMs, which has been particularly fashionable since the release of ChatGPT, but also more broadly in many other areas ranging from forecasting to option pricing, and I’ll try to go through a few of these which were discussed at QuantMinds.
AI from a broad based perspective
As part of the plenary session on the first day of the main conference there was a broad based panel on the impact of AI in investments featuring Theodora Lau (as moderator – Unconventional Ventures), and panellists Stefano Pasquali (BlackRock), Chandni Bhan (Wise), Nicole Konigstein (Wyden) and Yehuda Dayan (Citi). Pasquali noted how AI could help with many different areas, whether it was generating more signals for portfolio construction but also in terms of use cases such as compliance and trade monitoring. Whilst AI isn’t news, and there’s a bit of marketing about the topic, there is currently no escaping it, Bhan suggested. There had been an explosion in data, and it was possible to scan many different data sources, whether it was financial statements social media, geopolitical data and so on. Increasingly, there market events are becoming more interconnected. Konigstein also flagged how the recent executive order from Joe Biden on the subject of AI (see FT: Joe Biden moves to compel tech groups to share AI safety test results). On the subject of data versus the models, Dayan noted how whilst new technologies has created a level playing fields in some instances, data was important and could be a moat, even if the code itself was open source. Indeed, one thing I’ve noticed is that we’ve seen is many examples where libraries are open sourced, eg. TensorFlow, PyTorch etc. however, there has (unsurprisingly) been less of a willingness to open source data.
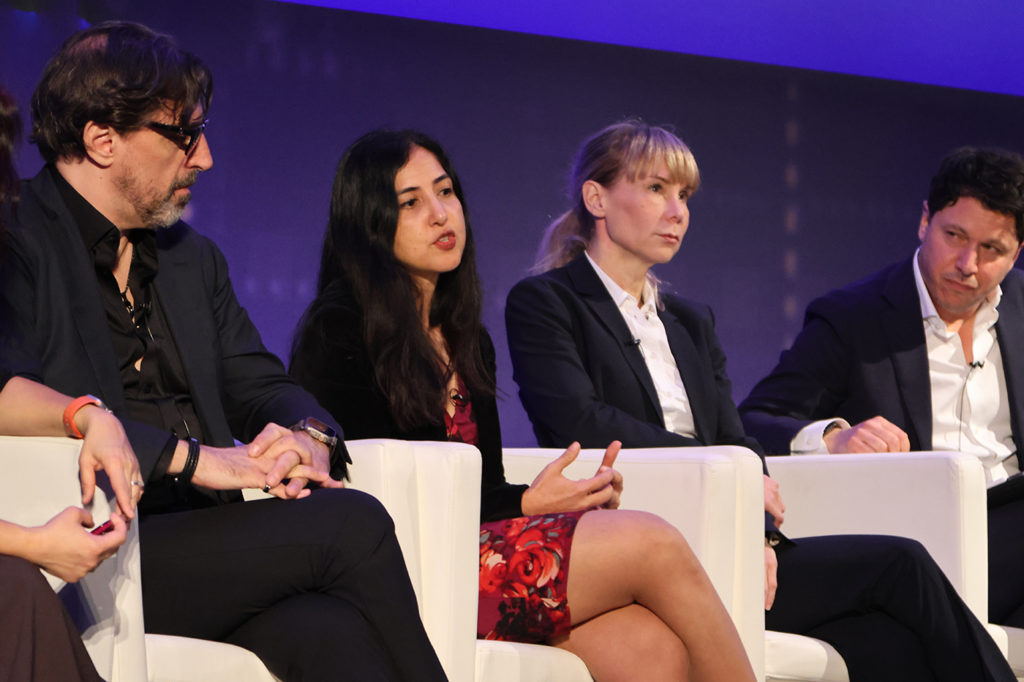
GANs
One place where generative models cropped up was in Rama Cont’s talk. There are a number of different ways to do VaR calculations. We can look at historical returns data and we could also also apply a Monte Carlo approach, for example assuming that our underlying follows a geometric Brownian motion. However, one thing we might miss with these approaches is to capture tail risk scenarios. Rama Cont’s presentation “Tail-GAN” discussed how to use generative adversial networks to simulate more realistic scenarios which would capture these tail risks to alleviate this issue (see SSRN: Tail-GAN – a generative model for tail risk scenarios).
LLMs and NLP
One of the most difficult things historically when doing natural language processing is the identification of keywords or topics to filter text that can be relevant for particular themes or assets we might wish to trade. In some instances, it is fairly obvious what the keywords are likely to be, but in other contexts it can be quite challenging. In practice it does end up being a laborious process with a bit of trial and error, directed by some level of domain expertise.
Vivek Anand and Ganchi Zhang (Deutsche Bank) discussed how AI could impact the future of investing, noting how it can be useful in a number of areas from asset allocation, risk management, stock picking and also trade execution. They also discussed how LLMs could be useful for helping with the problem of finding keywords/topics, whilst also being able to provide the explanations to the user for why these keywords/topics can be relevant.
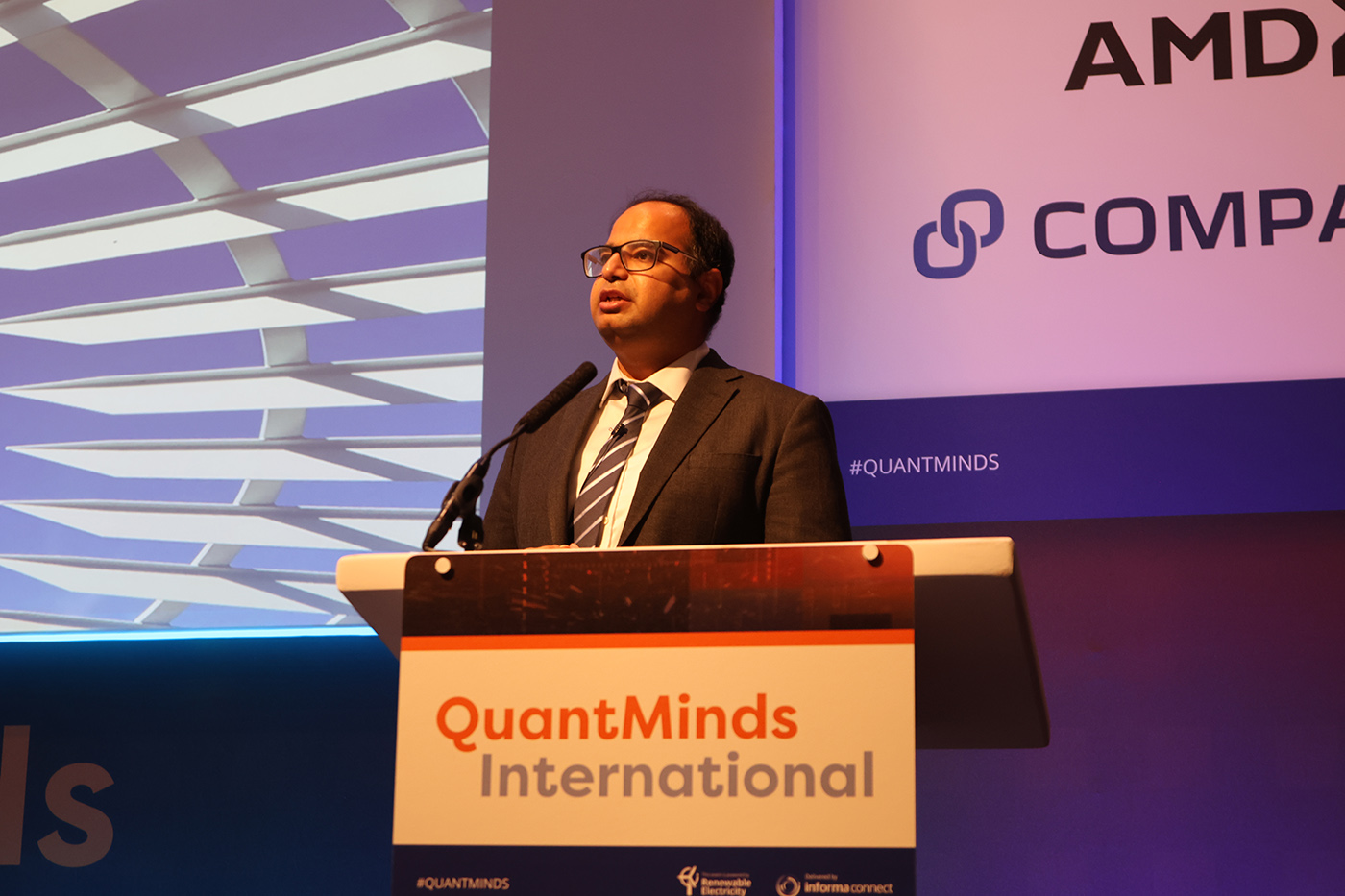
One interesting way to look at LLMs was presented by Alexander Sokol (CompatibL). He noted that we could view LLMs as like Monte Carlo, where LLMs are numerical algorithms for simulated token probabilities. Indeed, just as LLMs might have unpredictable output, where the answer could change between runs, this is something we also observe in quantitative finance (eg. Monte Carlo pricing). In essence LLMs map text to meaning (ie. a point in latent space), and basically compress a giant number of inputs into a relatively small latent space. Given sentences are so densely packed, you can do “interpolation” between them.
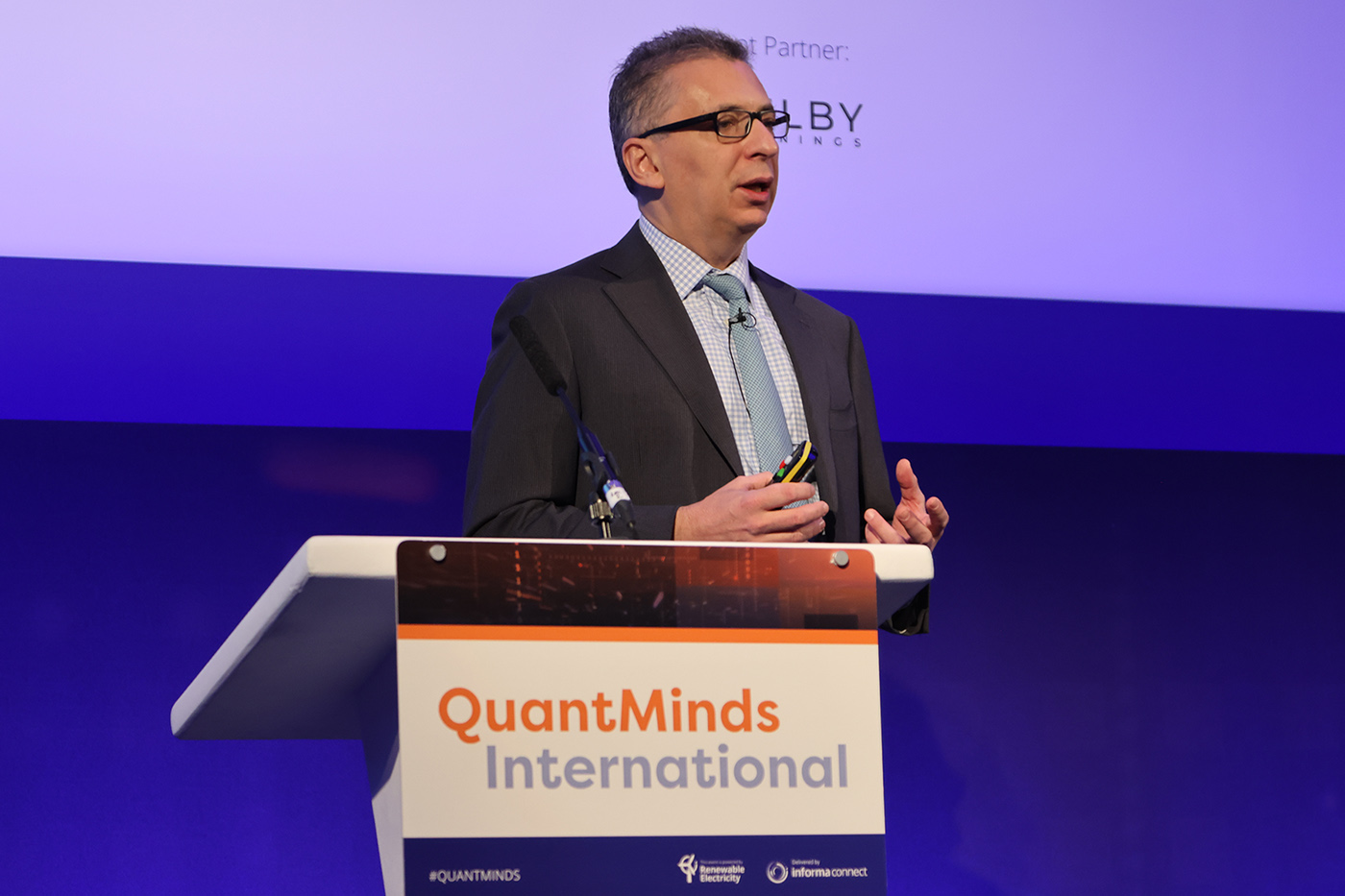
ESG and DEI
ESG and DEI (diversity, equity and inclusion) featured prominently at the conference both from a quant perspective, but also from also from the perspective of women in quant finance. In Eunice Zhan’s talk (Fudan University), she discussed how the signatories to pledges around responsible investments has increased over the years. She looked at ESG from the perspective of short sellers, noting how they were less willing to short stocks with high ESG rating, in essence that “overpriced” stocks which had high ESG ratings tended to be less shorted. The rationale is that socially responsible investors tend to be more patients towards companies which are “good citizens” (see SSRN: ESG Preference: Institutional Trading and Stock Return Patterns).
Focusing on the E from ESG, was Grigory Vilkov (Frankfurt School of Finance and Management) who discussed the pricing of climate change exposure, and a comparison between understanding the climate risk premium from realised returns versus from more forward looking data (eg. options markets). He noted that we are not yet in equillibirum yet when it comes to pricing these risks, and that the climate risk assessment came in two parts. First, there was the physical risk assessment, and second there was the transition risk assessment, which was essentially the part that humans could risk manage (see SSRN: Pricing Climate Change Exposure).
Looking at markets from a G perspective, Yehuda Dayan and Andreas Theodoulou (Citi) talked about using alternative data to quantify a companies performance from a diversity, equity and inclusion perspective. They noted how DEI was becoming more important looking at Google Trend searches over the years. For understanding the DEI of each company, they used alternative datasets primarily from online employee reviews data. They noted that DEI signals they generated were strongly associated with innovation, company performance and financial performance, and that the effect was higher on a relative basis.
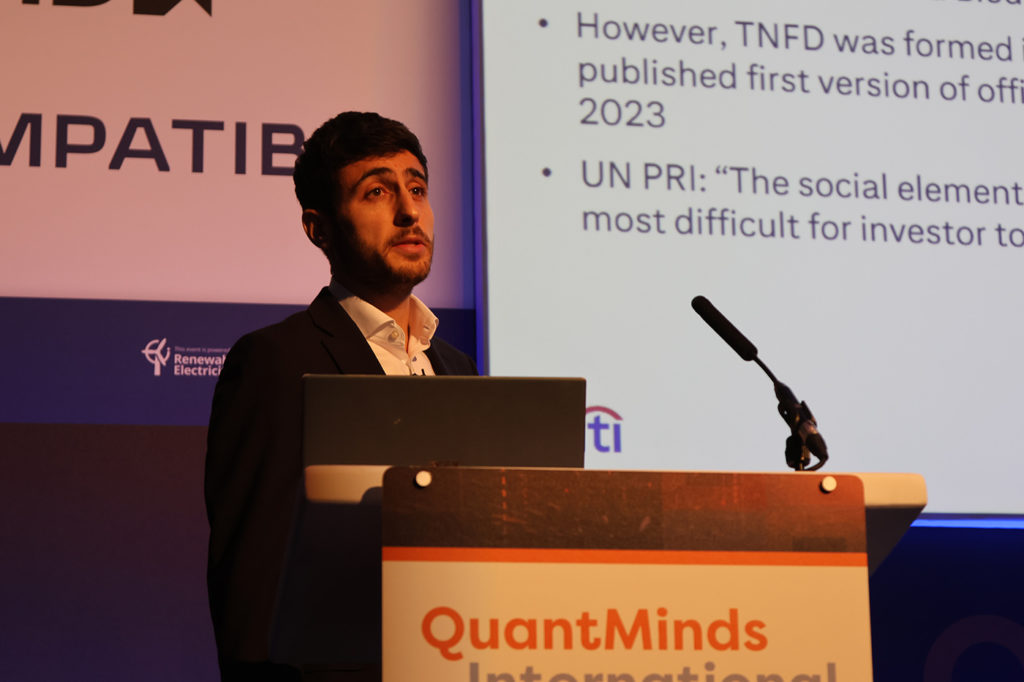
There was also panel on women in quantitative finance during one of the main plenary sessions, with Diana Ribeiro (Citi), Blanka Horvath (Oxford Uni), Leila Korbosli (UBS), Wafaa Schiefler (JPM) and Svetlana Borovkova (Vrije Universiteit). It’s pretty obvious to anyone working in quantitative finance that the representation of women is far less than men. It was noted in the panel however that at least a junior levels, the representation is getting a better. However, at higher levels such as managing director, it is still comparatively rare to see female quants. The true test of whether representation has increased will be in the coming years, whether the increase of juniors will translate into more women in senior quant roles. There was also some discussion about the differences between academia and industry when it came to female quant representation, although in the end there were disagreements about this, as noted by one of the panellists, the grass is always greener on the other side.
Signatures in finance
In recent years there has been more research in the area of signatures. The signature of a path is a collection of iterated integrals, that can be used to detect systematic patterns, for example in time series. We can use them to extract features that can be used in machine learning. Bruno Dupire (Bloomberg) gave a number of examples of where signatures could be used in finance, ranging from approximating payoffs and also in the context of deep hedging.
Blanka Horvath (Oxford University) and Owen Futter, looked at using signatures from the perspective of using signatures in the context of creating and end to end optimisation when forecasting returns. This would allow for optimal application techniques like volatility scaling, using EMA to generate signal inputs etc. which are traditionally done in a more adhoc/discretionary ways.
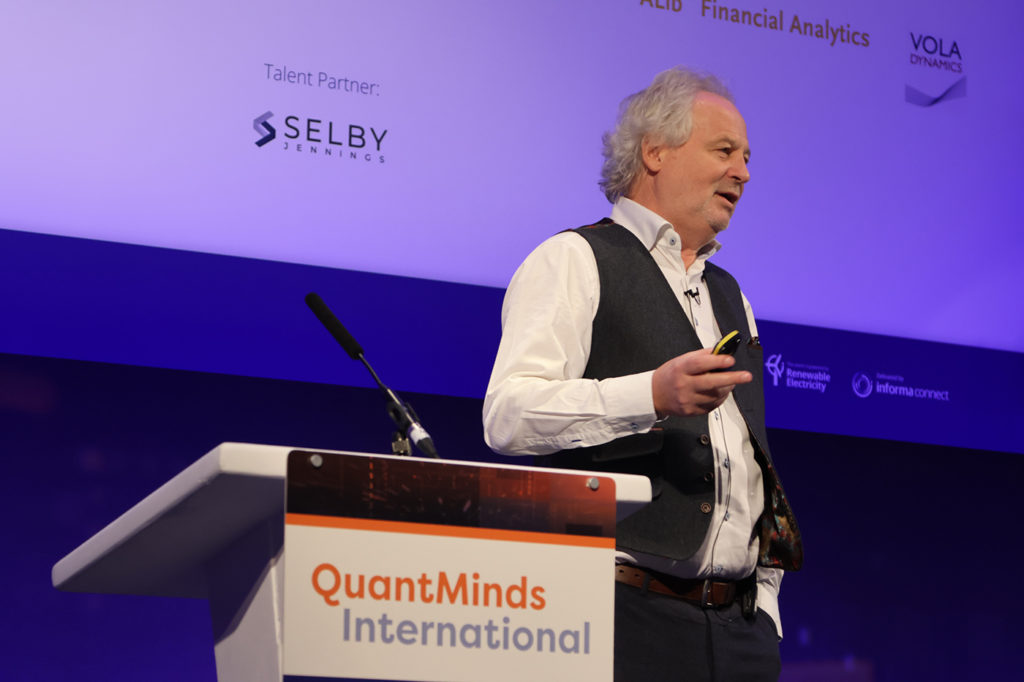
Marcos Carreira meanwhile discussed signatures from the perspective of building up rates curves, in particular in the Brazilian rate market. There are many challenges when looking at the term structure of a rates curve. Some points might be more liquid, whereas other points might be slow to update (given lack of quotes or trades). You can also view the rate curve from a time series perspective as opposed to at any point in time from a trader perspective. Risk managers might be looking more at historical data, when computing risk metrics. Furthemore, many of the points of the curve often end up getting bundled up together. Marcos showed how signatures could be used to give a hierarchical representation of the path, comparing original paths vs those reconstituted from signatures.
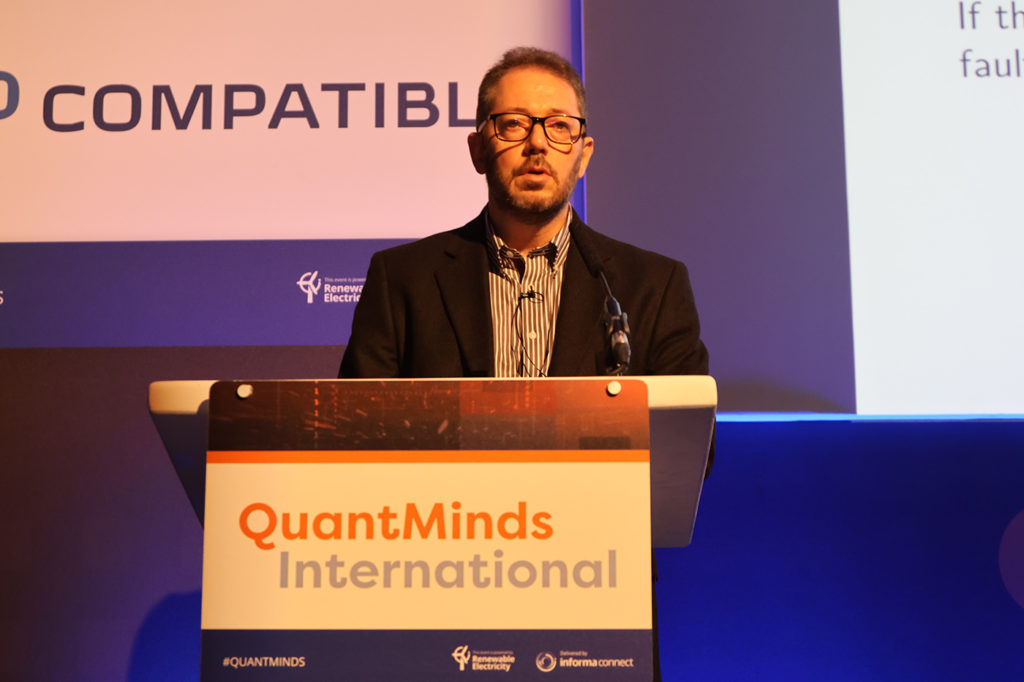
Quantifying market impact and using deep learning in short term pricing prediction
Last year at QuantMinds, Robert De Witt (Bank of America) presented an approach for using deep learning in the context of price forecasting for short term equities. This year he and his team returned, to give an update on the research, and also to show real life results from trading. In particular they discussed the use of Transformers to encode time series data as part of the forecasting process. They are mentioned how a probabilistic model helped them understand where the accuracy of their forecasts could be highest. It is interesting to see how deep learning is moving into a live trading environment, in particular in the context of algorithm trading, where there is a lot of data available to trade these types of models.
Zoltan Eisler talked about the measurement of trading costs. He noted it requires a lot of data to reliably measure costs. In practice many firms will outsource execution to the sell side. His objective was to speed up learning about trading costs to build models that could be used with more readily available datasets without the need for very expensive resources.
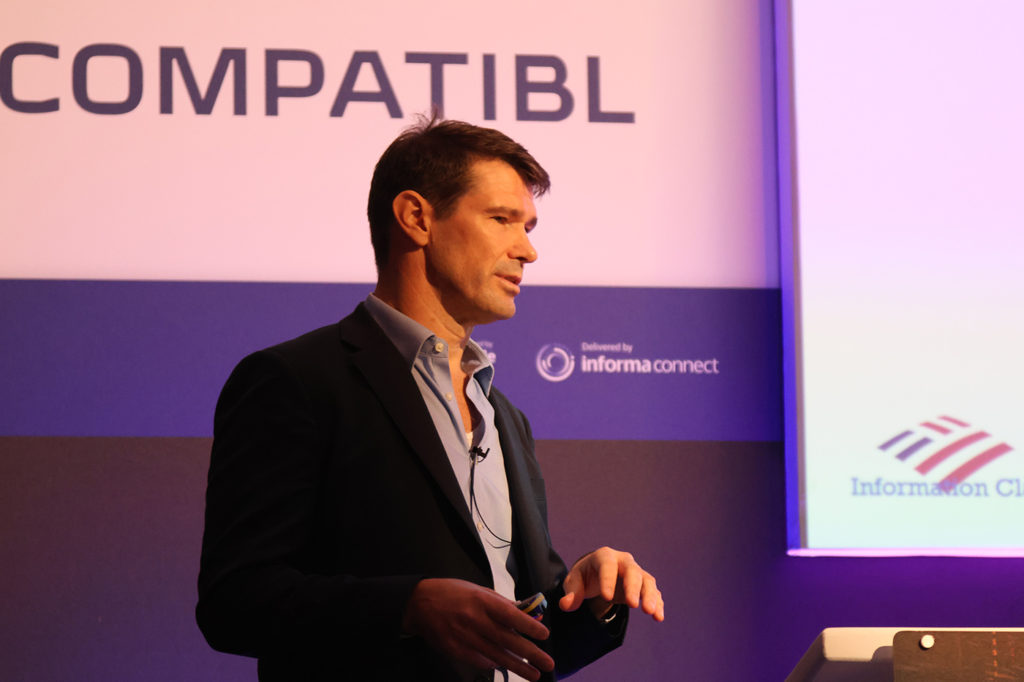
Macro perspective on quant
Traditionally, quants have been more focused on equities from a trading perspective. Indeed, there are many more datasets available for equities, and the asset class became mostly electronic a lot earlier than macro. In macro space, there is still a wide discrepancy for “electronification” with some assets like FX spot trading mostly electronic, whilst many others are still mostly manual, but transitioning.
There was a talk from Joe Hanmer (Fidelity) on factor investing from a fixed income perspective in EM sovereign bonds. He addressed many of the difficulties from the approach in particular from a data availability perspective, and how it needed a lot of work, in particular to build up curves for these instruments. He split up the task into differentiating between the issuer factors and also bond specific factors. The issue factors included looking at things like fundamentals, valuation and sentiment, with a data driven approaching, looking at inputs including growth and inflation. Whilst, it might be fairly common to see such approaches for equities, it is still (relatively) rare to see it being applied to credit. Probably one reason is the complexities in setting up the problem individually, constructing a curve etc. and addressing the data availability problem. It definitely looked like a promising area of research.
Richard Turner’s (Mesirow) talk was about time series more broadly, with an application to FX forecasting. He discussed using wavelets as a way to represent time series in such a way to help understand different drivers over time. The general approach was to add an additional step of wavelet representation as part of the forecasting pipeline. He also talked at length about using fractional differencing as one of the transformation steps when doing forecasting. The idea behind fractional differencing is that it enables you to difference a time series as little as possible such that it does not lose its memory, but at the same time, making it stationary when inputting it into the regression. He showed results which indicating that adding the wavelet step to the forecasting process helped to increase the accuracy of hit rates when forecasting FX.
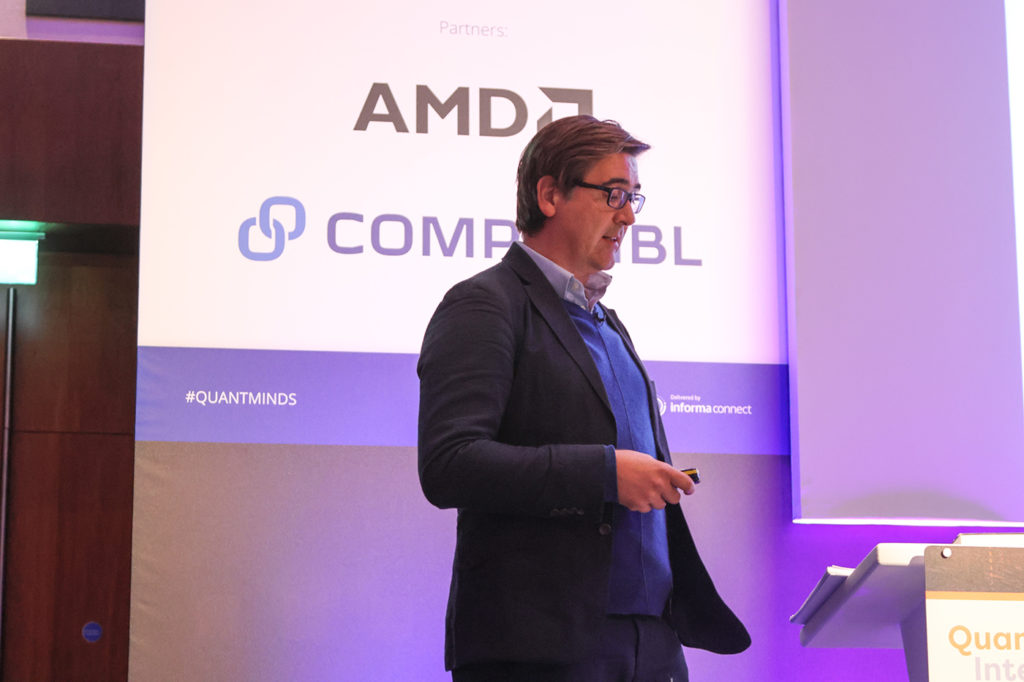
Also sticking to the macro theme, Alexey Ermilov discussed how to source alpha in macro space, beyond the traditional factor of trend following. He noted how global macro based strategies were investible across the major asset classes, including equity, FX, commodity, interest rate derivatives etc. However, there were differences in execution between these asset classes, where as listed products and FX were easier, interest rate swaps were more challenging, and execution couldn’t be fully automated there.
Global macro based strategies tended to be independent in the tails. He noted that it was possible to possible to learn from the approaches of discretionary macro, notably in terms of trading around economic events, but also more broadly trading based on fundamental analysis, using inputs such as looking at recent vs. historical levels in price, macro forecasting and a look at market mispricing. There were of course complexity in macro data, such as the issue with vintages and point-in-time economic data. You might need to “pool” signals, but also you needed to be careful not avoid individual effects on particular assets.
I appeared on a panel discussing the inflation outlook with Hamza Bahaji (Amundi), Alisa Rusanoff (Crescendo Asset Management), Osman Colak (ANZ) and Jukka Vesala (Nordea). We discussed a different topics, ranging from the types of products available to express an inflation view, to also the type of information you could extract from these products, including inflation derivatives. We also touched upon the difficulties with inflation forecasting, noting how central banks have found it difficult in recent years, given they didn’t anticipate how long the inflation shock would last. Later, I also presented separately discussing various machine learning techniques you could use for forecasting inflation, highlighting some of the work we’ve done at Turnleaf Analytics, which I cofounded with Alexander Denev. Inflation forecasting certainty isn’t a solved problem! However, we can use alternative data and machine learning techniques to improve upon more traditional approaches to the topic.
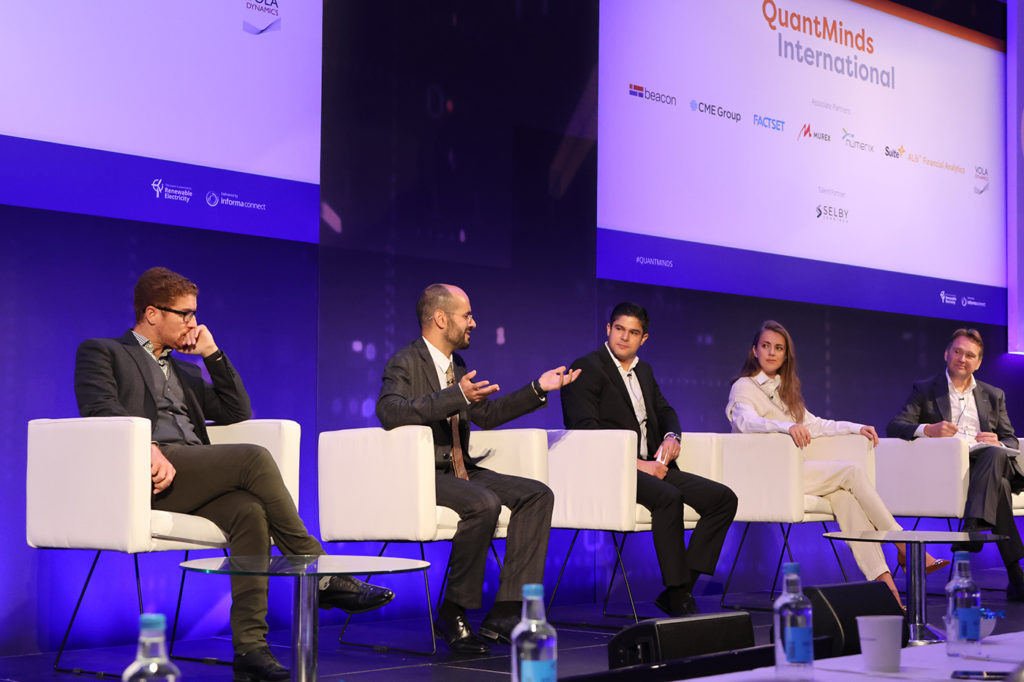
Conclusion
I remember a decade ago when I came to Global Derivatives, as it was then, noting how it seemed that many of the problems in quant space seemed “solved” at least those from an option pricing perspective. I was of course wrong, and the industry has moved on since then. Quants (and data scientists) are now working in many different parts of the finance industry, and there is a lot more research work left to do for us quants! The increase in computational power, coming at the same time as the greater availability of data and more advanced models, have opened up the quant perspective to many financial problems. It was interesting to see such a diverse array of quant topic tackled at QuantMinds, and to see how machine learning has become a core of the quant toolkit. Even a few years ago, much of the discussion was machine learning from a research perspective, whilst today you can see it in production in many areas. Let’s see what QuantMinds will be like next year, will LLMs still feature as brightly, or will there be totally new use cases from the machine learning sphere by then?