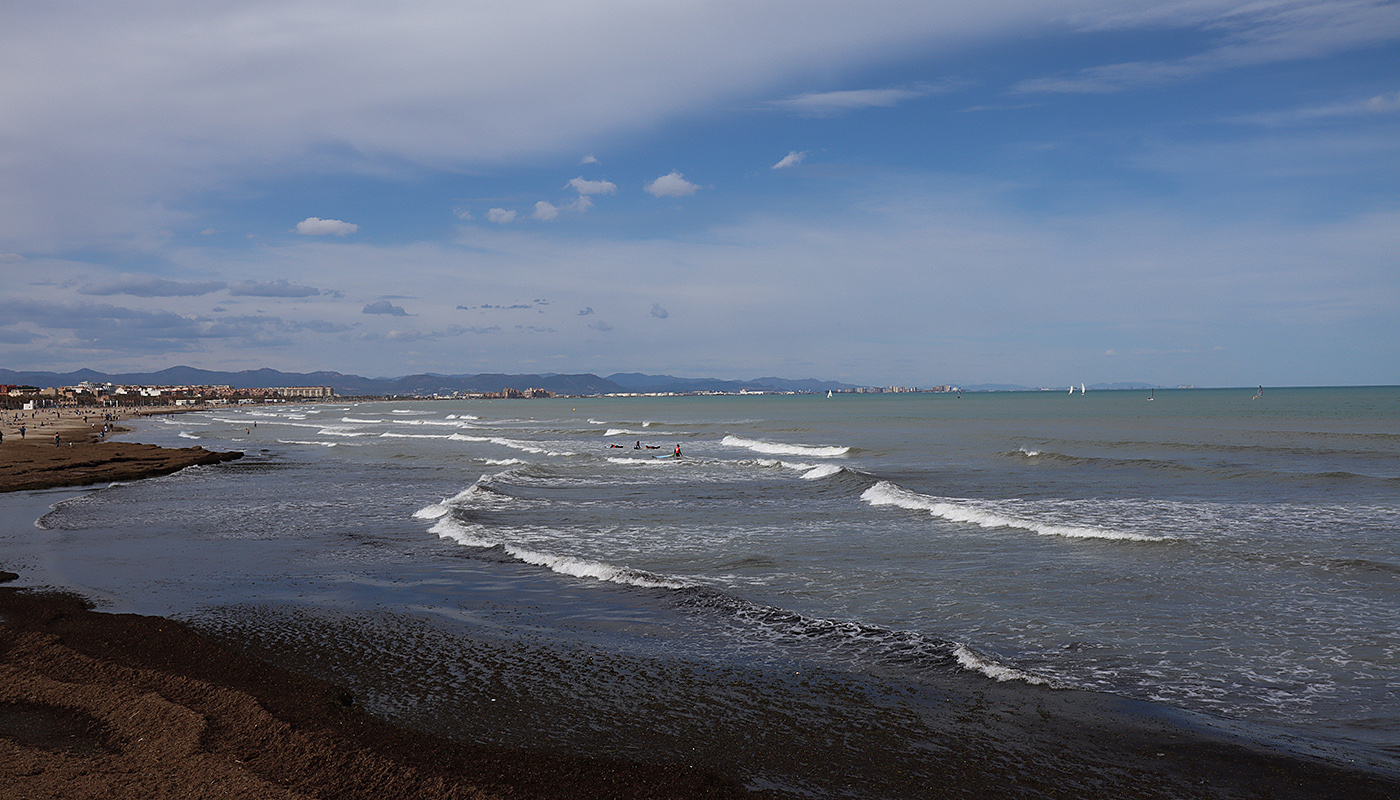
There are buzzwords and there are buzzwords. The buzziest (if that indeed is a word) of buzzwords in technology is that of machine learning, whether it’s using machine learning to improve image recognition, natural language processing etc. Although, I’ve got to admit, there’s still a long way to go… I still repeatedly get advised to apply for FX roles on LinkedIn, which are actually special effects (FX) animation roles, as opposed to F(oreign) E(xchange)!
Within finance, discussions on machine learning have increased too. There has for example been the publication many books on the subject such as Machine Learning in Finance by Dixon, Halperin and Bilokon. At most quant conferences, it is notable how machine learning has become a more important part of the discussion, and how it appears in many different contexts, whether it is in analysis of market microstructure, outlier detection, option pricing etc. Of course, as a domain, applying machine learning in macro (and finance more broadly) has been more tricky than elsewhere. If you are doing, for example, image recognition your training set and test would (hopefully) have the same distribution and we can make the assumption that the data is stationary (cats don’t change how they look over time!). In finance, we have to contend with data which can often be nonstationary.
Macro has perhaps been slightly slower to look at machine learning compared with other areas of finance. Although, this does appear to be changing. Indeed, I recently attended an advanced analytics conference organised by the Bank of England on macroeconomic policy, where machine learning featured in most of the presentations and posters presented, ranging from forecasting to discerning signals from alternative datasets compromising of text, video etc. At Turnleaf Analtyics, which Alexander Denev and I recently cofounded to forecast inflation, we are also using machine learning.
In macro, the relationships between variables may not always be linear. Hence trying to use machine learning models can be attractive when doing macro forecasting to capture these nuanced relationships. At the same time, there are of course challenges. There is still the need to go through the process of finding data and preprocessing. If we don’t have the right data, no matter how we model, they’ll be problems. Perhaps, it’s even more important when we’re doing machine learning, because many newer techniques can be data hungry. Indeed, our experience at Turnleaf Analytics, is that we have spent a large amount of time on preprocessing data and also finding additional alternative datasets, many of which need to be structured as well. Unfortunately there isn’t a short cut and it is important to understand what the data means in context with domain specific knowledge.
When using the any sort of model, whatever it is, we need to understand how the model works. Some models might be more suitable for a particular problem than others, and for that we need to understand the theory behind a particular model. What might but a good model for some situations might not be appropriate for others. At the same, sticking to very simple models might not necessary capture the dynamics we want (underfitting the data). Equally, a model which is too complicated for the problem at hand can result in overfitting (see bias-variance trade off).
We also need to strike the right balance between explainability and performance of a model. After all, in macro, understanding a forecast is key. If it is a fully black box, and we haven’t any developed tools to explain our results, it can be more challenging to utilise the forecast. It’s also worth pointing out is that even simple techniques like linear regressions can become less explainable in any case and we increase the chance of overfitting, if end up with large numbers of variables.
The world of macro is changing. Machine learning and alternative data are becoming more important components of understanding macro. At the same time, the basics of cleaning and preprocessing data are just as important as ever (perhaps even more so), as is understanding what type of data is important and the models you are using.