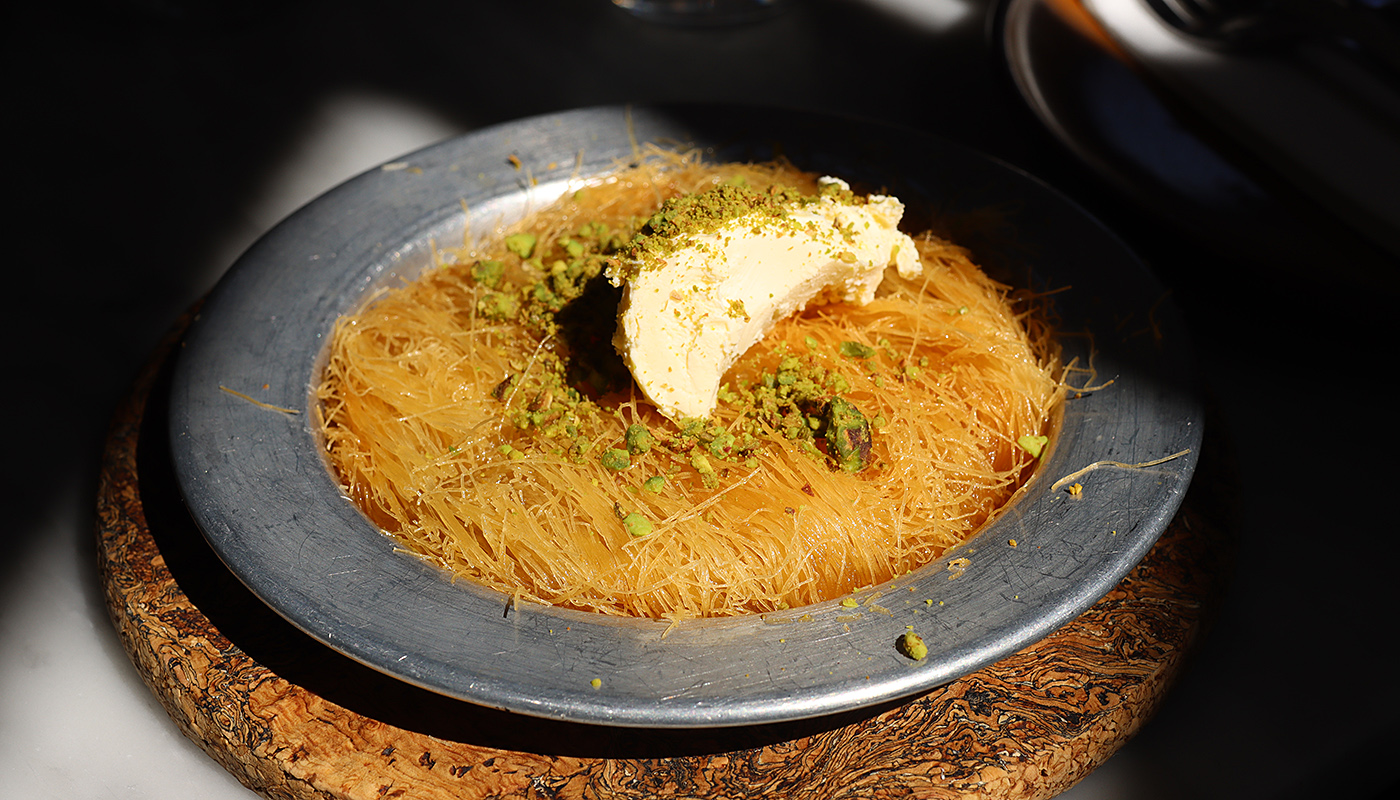
We all want to do the cool stuff. We want to go on holiday, but maybe we’d like forget about the time it takes to get there. We want to go a nice popular restaurant, but maybe we’d like to forget about how the time it takes to queue we need to queue. We want a burger (or at least I do…), but maybe we’d like to forget that crazy little thing called fat content of a burger. I could probably go on and on, giving citing similar examples. I suppose all these examples can be summed up by the cliche of “no pain, no gain”.
In finance, it’s exactly the same thing. We want to get to answers. However, in order to do any sort of financial analysis of markets, we need the clean data. Getting your data into a form so that it can be analysed is very time consuming. One important element is having appropriate identifiers. We often have multiple datasets that we need to join together.
Let’s say we get market data from a large market data provider for equities, and we also have some alternative data for equities (eg. proprietary equity earnings estimates). We need to make sure that we end up having a common set of tickers between the various data providers, so we can combine these disparate datasets. For traded assets, there are now common standards for instrument tickers. We have a number of different standards for tickers, including ISINs, FIGIs (originally Bloomberg Global Identifier) etc. There are also proprietary mappings, such as RICs (eg. EUR=), which is used by Refinitiv.
However, traded instruments are not the only things, where common identifiers are useful. Financial models may have many different sorts of data. There’s also the open standard PermID, which provides mappings not just for instruments, but also for example people. Let’s say we have multiple news datasets, articles will not only be tagged for tickers which are mentioned, but also entities such as people. If we want to join together multiple news datasets at an entity level, and the mapping for entities are totally different, we’ll need to create our own mappings, between them.
At Turnleaf Analytics, which Alexander Denev and I cofounded to do inflation forecasting using machine learning, we use many different sorts of datasets. These datasets all need to be combined together. Hence, managing tickers becomes very important. These datasets range from traditional, such as market data and macroeconomic data to less commonly used alternative datasets. Macroeconomic data can be particularly challenging to use. There will be many different versions of the same dataset (eg. different vintages, seasonally adjusted or not etc.). There isn’t often a common approach to tickers across different economic data sources (eg. different central banks and national statistics providers).
Creating a properly managed system of tickers takes time. However, it becomes a lot easier to manage our different datasets, and ensure that we can join them properly together, once we have such a system. Ideally, if we can take advantage of existing standards like FIGIs and PermIDs that will be helpful and save us time. We might also want to create our own shortcut ticker names for other types of entities to make them easier to work with for human users. For investible tickers like equities, we already have common “shortcut” ticker names, eg. AAPL for Apple stock. For example, it’s all very well . Data providers can help in this process of ticker management by the way they categorise the data. Yes, all this ticker management sounds like it’s a big investment in time, but it’ll be worth it.