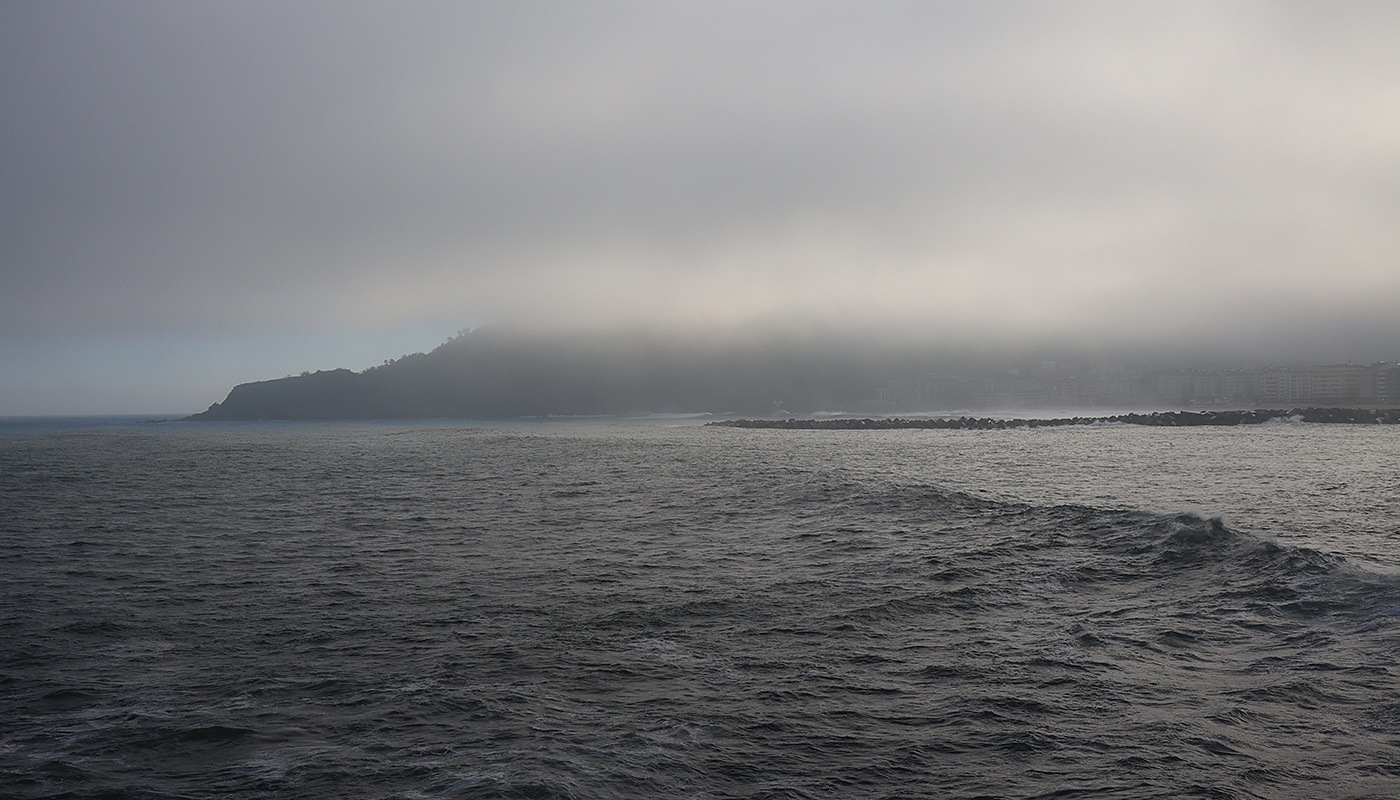
There are some ingredients that are pretty common. Just because they’re commonly used, does not make them unimportant. If anything, they are the most important when it comes to food. Without common ingredients like flour or oil, it’s going to be quite limiting. By the same token, very rare ingredients are not necessarily the most important. Sure, a hint of truffle (or more likely truffle oil) gives food a different and somewhat unusual flavour. However, you could probably get by, without ever having any truffle in your food. It’s all about mixing ingredients, whether common or rare into that perfect mix.
Data comes in many forms. Just like food, you’ll have your rare (or truffle!) datasets, many of which might be alternative data. You would expect that any alpha in these alternative datasets to be more long lasting. By contrast, with common data, which are used more commonly, we might expect that they give us more beta than alpha. After all, if it’s common data, more market participants will use it, and there’s a higher chance that any alpha has already been harvested.
Does this mean we should prefer to use alternative datasets instead of more commoditised and common datasets? Not really. Just because a dataset is commonly used, it doesn’t mean it can’t give you insights. It’s just that other folks in the market are likely to gain similar insights! If you ignore these datasets, you might end up getting blind spots, which most others in the market can see.
Furthermore, as Alexander Denev and I discussed in The Book of Alternative Data we very often want to combine together datasets together in a model. These can be a mix of alternative data and more common datasets, such as price data. It might be the case that any one dataset or factor might not given us a lot of power. Indeed, this approach of mixing datasets is the approach that Alexander Denev and I have taken in our new venture, Turnleaf Analytics, for forecasting inflation.
Alternative data can help us when it comes to modelling financial markets. It can give us insights which are less likely to have been harvested by other market participants. However, importantly, we should combine alternative datasets with existing datasets. We can combine together many signals from different datasets, which may in isolation be weak, but on aggregate can be strong.