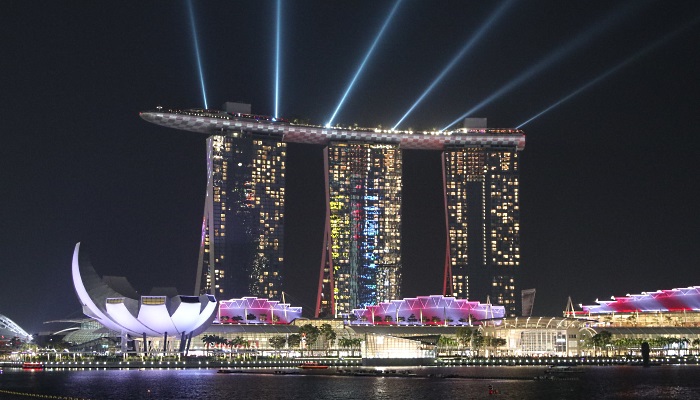
I’ve never met anyone who just eats tomato ketchup. Ditto for anyone whose diet simply consists of lettuce. The same goes for those whose intake consists exclusively of gherkins. Why? First, such a monotonous diet is unlikely to be healthy, and a sure way to deprive yourself of healthy nutrients. Second, devouring tomato ketchup (or any of the above) is likely to get very boring after a while. The whole point of something like ketchup is of course to use it as a condiment. It accompanies food, such as.. burgers! Lettuce can be eaten on its own, but it’s much nicer in a salad with some dressing. Even something like bread, is well, nice by itself, but so much better when it’s part of a meal.. like a burger again (ok, you can probably guess the burger theme weaving its way through this article..)
The same is true of data for investing. You can look at specific datasets in isolation, like trying to eat ketchup on its own. It is possible you might find something amazing, a signal that generates significant alpha. For example, just by looking at price alone you can get some great market insights. However, very often price data in isolation is often no longer enough for many situations (there are exceptions!). Hence, as a result these days, there’s a real buzz around alternative data. Alex Denev and I are currently coauthoring a book exactly about this, The Book of Alternative Data (Amazon pre-order here), which will be published in early 2020. The idea is that looking at less commonly used alternative datasets could give you an edge, that other market participants don’t have. However, very often simply looking at a single dataset can be tricky. In many instances, there might not appear to be a strong signal from it for trading. The whole point of adding a new dataset to your data arsenal is not that it works well in isolation. It is supposed to add incremental value to what you already know about financial markets.
Let’s say we have an alternative dataset, which can help you predict market trends very well, and a trading strategy using it has decent risk adjusted returns. Then you look at your portfolio, and lo and behold, you already have a trend following strategy based on price data.. and guess what, your alternative dataset has a massive correlation with it. Suddenly, the fact that your “alternative dataset” works very well alone, becomes a bit irrelevant. You are just reproducing an existing strategy, but with a more expensive dataest. It isn’t telling you anything extra about the market, you don’t already know. In other words, we want our new dataset to tell us about the data we don’t know.. not about the data we already have!
By contrast, we might test another alternative dataset, which in isolation appears to have a weak signal. Some folks may simply therefore simply ignore it (which is probably good for us). However, when combined with your existing strategies (let’s assume trend following), it provides incremental value, and significantly improves your risk adjusted returns. First impressions can sometimes be misleading when it comes to evaluating a dataset. Obviously, this all means that when we’re testing any alternative datasets, we need to think laterally, about which other datasets and strategies to combine it with and in particular how they might be complementary. It’s just like hiring someone, if you hire someone with your exact same skillset and experience, sure, it’ll let you scale out your work, but it’s also important to have a diverse team. A team with many different skill sets and a different approach might think of solutions, you would never have thought of.
So when it comes to data, just as with food, tomato ketchup is best consumed with a burger! It’s key to think laterally about how data can be combined, that’s where the alpha is.