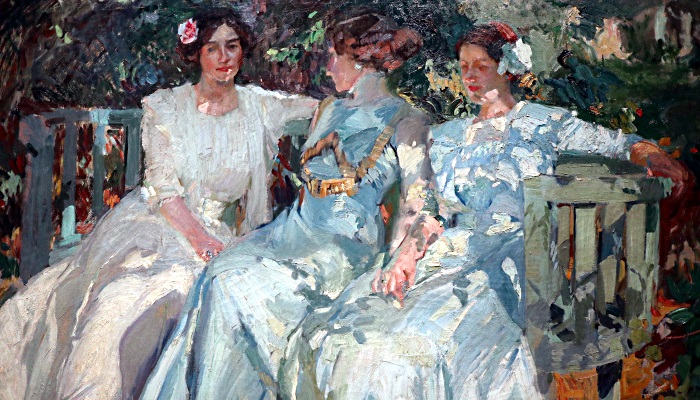
I had never heard of the painter, Sorolla, until I recently saw an advert on the Tube for an exhibition of his work at the National Gallery. This week, I visited the exhibition. What is immediately apparent from his work is the way he uses of light, and in particular sunlight (and perhaps it’s not a surprise that the exhibition was entitled Sorolla: Spanish Master of Light). The paintings have a particular ethereal quality to them, which is more apparent when you see the paintings in real life. The light brushstrokes to illuminate the paintings are not always in an obvious place like on the subjects’ faces. Instead, Sorolla chooses to illuminate the folds in fabric they wear. The effect can be quite different, depending on how close you stand to the painting. Further away, the light and the dark seem to merge. Close by it’s possible to pick out individual broad based brush strokes.
There is no set way to “paint”, each artist has their own style and way of interpreting the scene. I’m sure if another artist had painted the exact same scenes, it would almost certainly look different to what Sorolla has painted. That after all is the whole point of art, for the artist to interpret what they see and to express themselves. It is also clearly an artistic element in what an artist chooses to paint. A photograph may record a scene most accurately, but it is different to a painting! Of course, technical skills are necessary in order to put paint it on to a canvas, and indeed it takes a lot of time to hone these skills (and in many cases it might simply be impossible.. I’ll never paint like Sorolla for one!). However, technical skills in isolation are not sufficient.
When it comes to data science, a lot of the emphasis can be on the various techniques we can use to slice and dice data, and how to code. Of course, having a good understanding of statistics and being able to write code are very important, and these skills need to be learnt. However, in practice, before we even pick up our keyboard in anger to type, and write some Python for a particular project, it’s important to think about the artistic element of data science. What questions do we want to ask? What data can we use to answer these questions? Only then, should we start number crunching.
Even when it comes to actually answering the question and coding, often it can be an art too. Sometimes we might need to combine together different statistical techniques. Coding is also a creative process in itself. There’s a big difference between some code which works, and code which is elegant, scalable and maintainable. We often need to understand our domain too, which in my case is finance. This can help us articulate the question and also avoid mistakes. When developing trading strategies one common mistake, is not understanding liquidity and the market. Hence, stuff might look great on paper, but with reasonable transaction costs, it becomes totally untradeable. I could go on and on about mistakes that it’s possible to make, if we have no understanding of the domain, but it really is an important! If you have no domain knowledge, it’s worth working with someone who does have it.
Data science really isn’t a matter of pointing and clicking and getting an answer, which has been amalgamated through a plethora of data mining, without any real thought or direction. Yes, you need to learn the technical skills which take time and effort. That is a prerequisite, but it needs more than that. Data science, whether in finance or outside of finance, is about thinking laterally and creatively to ask the right questions! It’s just like trying to compare an Instagram filter to Sorolla’s painting, and somehow equating them… please don’t do that! Please do go and see Sorolla’s exhibition in London before it finishes in July!