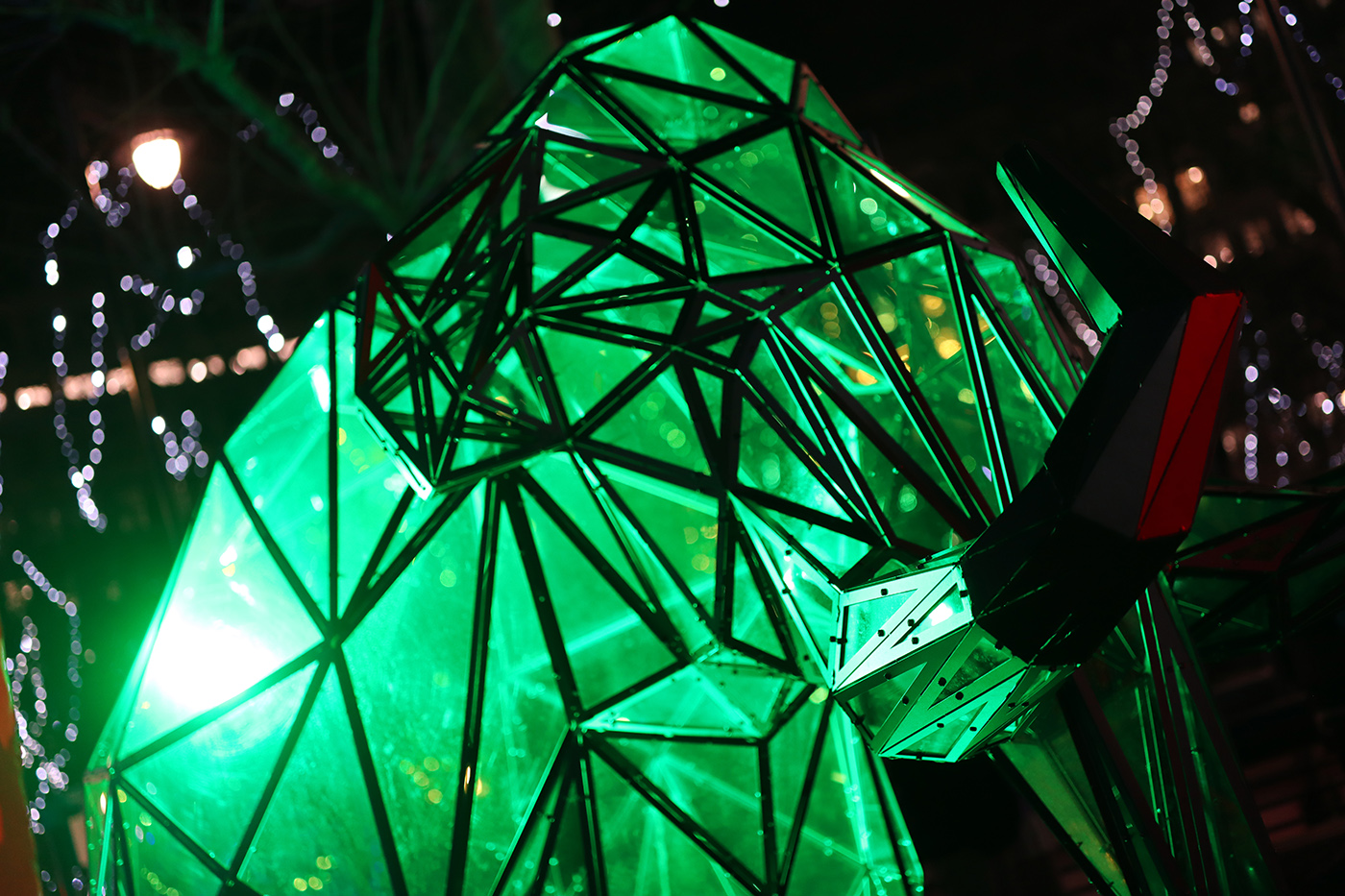
Harold Macmillan, the British prime minister between 1957-1963, was once asked, what was the most challenging thing he faced. His reply was “events, dear boy, events”. It may well be an apocryphal, and it has been suggested that he instead said “the opposition of events”. Politics is obviously not the only place, where events have an impact, it is also the case with financial markets.
Within financial markets, there are a multitude of repeated events, whether it’s Fed meetings or economic data releases. We obviously don’t know the outcome of these events, but the fact that similar events occur repeatedly at least allow us to study them in more detail. Furthermore, their timing is scheduled. There could be considered as “small data problems”. I recently went to a day of presentations at Cambridge University organised by their algorithmic trading society. One of the presentations was by Chris Longworth, of GAM Systematic, who discussed small data problems. He noted how they can be prevalent in finance. This contrasted to other areas, where Big Data was more prominent. One area is the example of computer vision, where we can use masses of data to train very deep learning models.
Many of the problems I’ve ended up facing finance have been small data problems. In particular, at present at Turnleaf Analytics, which I cofounded with Alexander Denev, we are forecasting economic data releases, focusing initially on inflation. Inflation prints are indeed repeated events, but they occur once a month in general for each country.
However, aside from repeated events, we also have to deal with rare events, or one off events in financial markets. In some cases, these events are unexpected in terms of their timing, such as COVID. In other occasions, their timing is known, but not their impact. We could for example include the Brexit vote of 2016 in this bucket. How can we deal with rare or one off events in a forecasting model? From the outset, we also need to be realistic, that some events are going to be totally unexpected Black Swans, which are by definition not predictable, but the market also faces this problem too!
Our objective may not necessarily be to forecast such a one off event, but such an event could impact whatever variable we are forecasting. If the event timing is known, we can try to include datasets which can help us understand them. In the example of Brexit, using implied volatility data over the event, would at least give us an idea for market pricing, even though Brexit had never occurred before in our historical dataset (the closest event to it was the Scottish independence referendum). It would give us an indication that GBP volatility is likely to be much higher over the event, which could lead us to trim risk. We could extract the implied PDF from the vol surface, to get an idea of the potential size of GBP moves.
For COVID, it would have been far more difficult, given that the timing was unexpected as well, and as the situation developed it was not clear how severe the impact would be. However, later on, datasets on COVID cases (which did not exist before) became very important for tracking it, and also alternative datasets such as Google Mobility, to understand the impact of policy decisions such as lockdowns.
In general, when running a model, we learn a lot about the problem at hand in real-time, and we should improve the model if we find new techniques and datasets which can improve overall performance. Improving the model though is somewhat different to using expert judgement for one off/not repeated events. Expert judgement is an overlay on top of a model based forecast if we’re trying to adjust for a rare event. The difficulty is such an expert judgement is of course subject to uncertainty and can change significantly, not only in the run up to event (where timing is known), but also after the event has actually occurred.
What is key though, is that any expert judgement which is made for one off events, should be clearly labelled as such. If we have a model and make expert judgements on top, we should clearly record what the expert adjustment to our model was. Over time, we should be able to understand and quantify the value of an expert judgement, whether is positive or negative. It makes our forecast more transparent, if you know what part of it was related to an expert judgement and what was related to a model.
Financial models are impacted by events. If an event is repeated regularly, even if the outcome is clearly different in each instance, the backtest of the model would contain information that could be useful in modelling that event. However, in one off/rare events it is far more difficult. We can try to apply an expert overlay to our model, but there is the caveat that any expert judgement itself is subject to uncertainty and it may not always improve our forecast’s accuracy. If we do go down the expert route, we need to be clear how we are adjusting the model forecast.