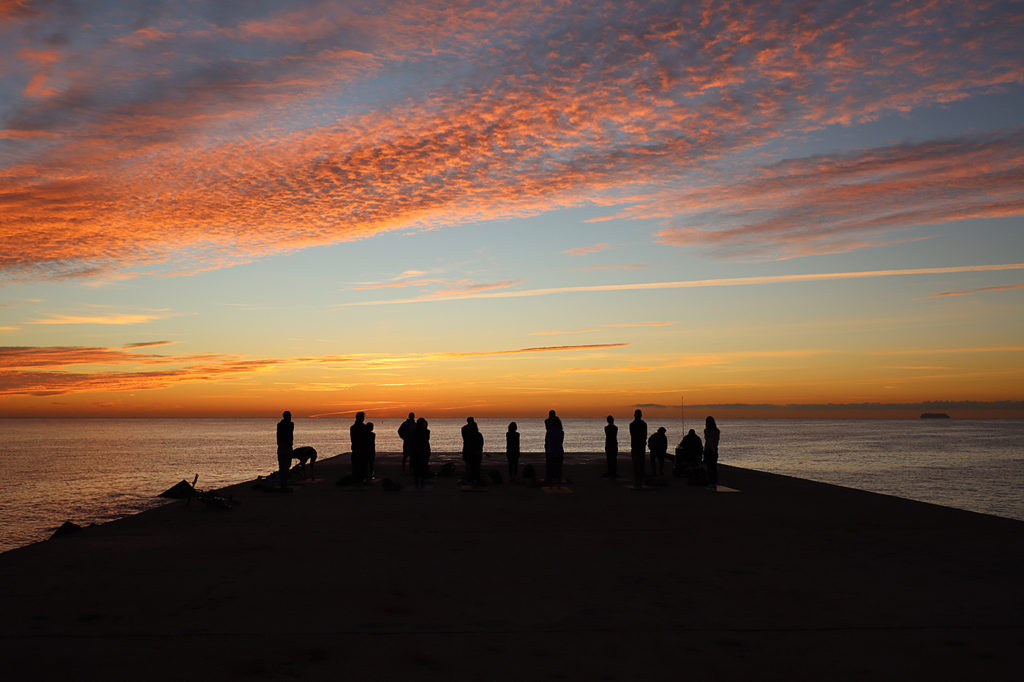
It’s been thirty years since Barcelona held the Olympics. Whilst I never visited the city before the Olympics, it’s difficult not to notice the impact of the event. You can see this in many areas of the city, such as in the seaside, where the beachfront was developed. The legacy of the Olympics in Barcelona was perhaps more lasting than it has been for many other hosts of the Olympics. At the same time, walking around the city, it is still very much a city of history and the city of Gaudi. Indeed, Gaudi’s creations remain the most well known symbols of the city, most notably La Sagrada Famila.
Barcelona was the host city for QuantMinds for the second year in a row. Last year’s event was held at a time when there were still many restrictions related COVID. This year, the event finally felt like it did before the pandemic, with noticeably more attendees than last year. In this post, I’ll endeavour to summarise my takeaways from the event. As usual there were a very large number of talks, and as such I can’t cover all of the ones I attended (and the many which are were not able to attend given that there were many simultaneous streams), but I’ll try to give my takeaways from the event.
The general trend in QuantMinds has been towards a more diversified array of talks. Of course, there are are still many discussions around more traditional areas of quantitative finance, such as option pricing,. However, these days, there are more events related to for example alpha generation. The topic of machine learning also featured prominently across many different areas, ranging from option pricing to NLP to trading strategies.
The event kicked off with the AI and ML summit day. The first talk was from Matthias Fahrenwaldt, from BaFin, discussing machine learning from a regulatory perspective. He noted that techniques from ML have been around from decades, covering areas such as regression, classification and data clustering. When comparing between classical statistical methods to those associated with AI/ML, there wasn’t a clear divide, but instead a continuum between the two approaches. Classical approaches used a smaller number of variables, with simple assumptions and this resulted in transparent models. In the ML approach, instead it relied on much larger datasets, and many parameters. Data quality is always an issue but became even more so with ML techniques. In a nutshell he explained that existing supervisory practices still covered ML.
Andreas Nardon’s talk discussed using machine learning in the context of portfolio construction and risk management. He noted the opportunities in terms of modelling market relationships in a non parametric way and also the challenges (such as overfitting, non-stationary data etc.). He gave a specific example from asset allocation and the use of decision trees, using a filter based upon VIX and S&P 500. One of the benefits he noted from decision trees, was that they were comparatively simple.
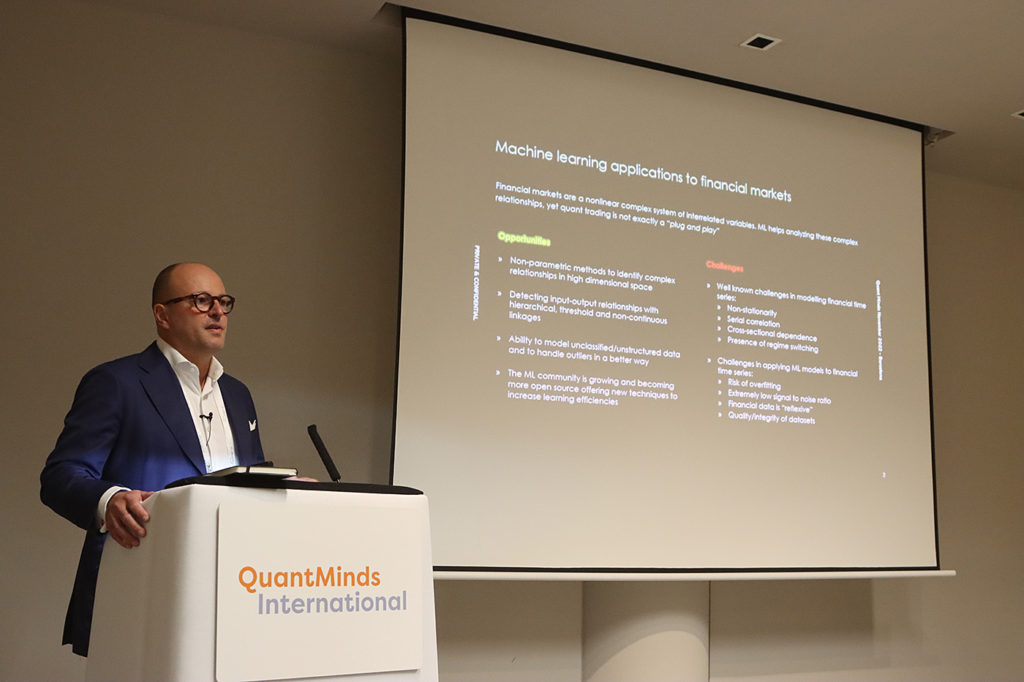
On subject of NLP, Caio Natividade and Ganchi Zhang gave an enlightening talk on the use of sentiment within the context of equity investing, in terms of generating alpha and also risk modelling. Text based approaches to understanding financial markets have largely focused on equities. However, NLP is also applicable for macro markets, albeit the approach in macro needs to be somewhat different to that in equities. From Sylvain Forte, at SESAMm, we heard about the use of NLP in macro and commodities, with use cases from a number of different areas including monetary policy. He noted how they use many different NLP algorithms, such as BERT etc.
Also on the topic of NLP, Ioana Boier, from NVIDIA, discussed large language models and how they could unlock new opportunities, noting the emergence of BERT and other similar models, with some tuned for finance. She discussed how these models could be trained, initially often involving a generic model and later fine tuned, for example through zero/one shot learning. In the later conference, there was also a presentation from Peter Hafez (RavenPack) on using NLP to understand labor markets.
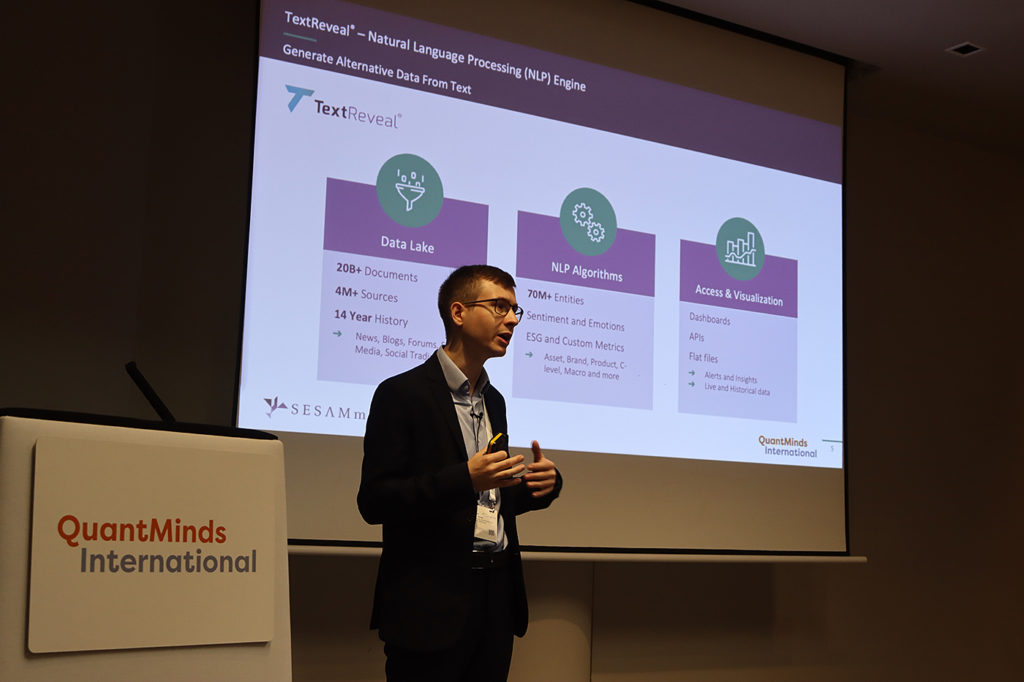
I also presented how Turnleaf Analytics, the firm Alexander Denev and I cofounded, approaches inflation forecasting, noting how machine learning can provide a way to capture non-linear relationships in the various input variables, which an approach such as OLS struggles with. I also followed up with a section of my talk discussing how to speed up Python in your tech stacks. Our work can be computationally intensive particularly when doing sensitivity analysis on our inflation models, hence, it is important to spend time optimizing code. I discussed techniques such as Numba (a LLVM) to accelerate Python code and libraries such as vaex that can handle calculations on very large datasets that do not fit in memory.
Svetlana Borovkova discussed using deep reinforcement learning to find strategies to delta hedge options, a subject which has been become more popular in recent years. The approach was to use P&L of the hedging as the goal in the reinforcement learning problem. She showed how the approach could reduce P&L volatility.
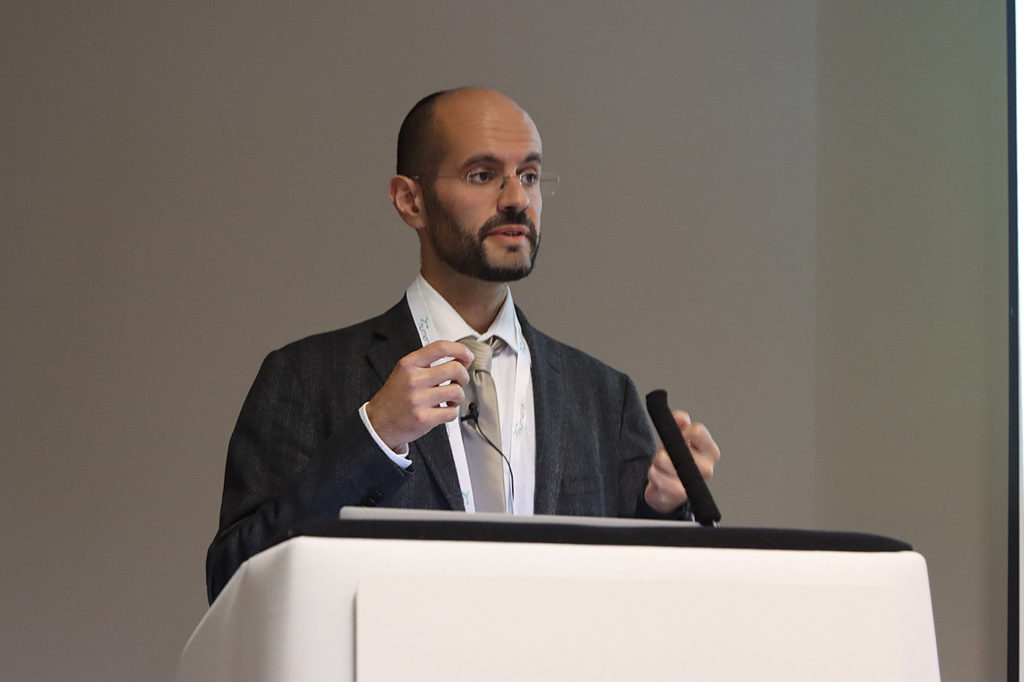
The bulk of the talks were over the main conference, which took place over the following 3 days after the summit. It included talks from very well known members of the quant community including John Hull, Bruno Dupire, Alexander Lipton and Rama Cont. This year, QuantMinds also honoured the legacy of Peter Carr, who unfortunately passed away earlier this year. The Peter Carr stage at QuantMinds was dedicated to him. I saw many of Peter’s presentations over the years at QuantMinds, which were also always amongst the most popular and he had a very intuitive way of explaining his research. I met him a number of times, and what I will always remember is that always had time for you, and it’s something that I will always appreciate.
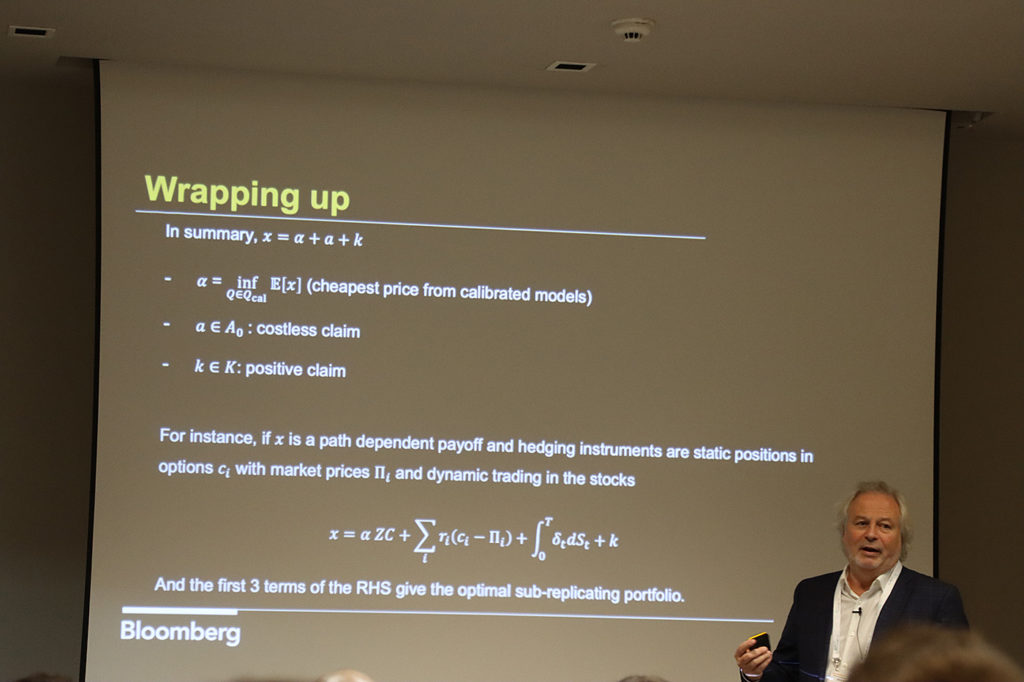
The main conference was opened by a presentation from Ed Altman on zombie companies, a term which often gets banded around in the popular press, but without much in the way of a definition. Altman explained that a zombie firm was an insolvent firm, which still functioned over a relatively long period of time, discussing their pros (ie. employment) and their cons (misallocation of funds). He discussed quantitative measures for identifying zombie firms, and how they varied between countries.
One of the major challenges facing financial firms, but corporates more broadly is how they can take advantage of machine learning approaches to add value. Cassie Kozyrkov from Google, gave a presentation on why businesses fail at machine learning and AI. She made a distinction between pure ML, eg. coming up with algorithms and applied ML, where domain specific expertise was needed. Often the skills between these two areas were not necessarily transferable. Some of the biggest opportunities were the fact that ML could be used to define those solutions, which were simply too difficult to explain otherwise. The threats included bad data quality, and a lack of leadership. Trust in models could be gained in these models through testing. Rather than asking how does it work (about a model), instead we could ask does it work?
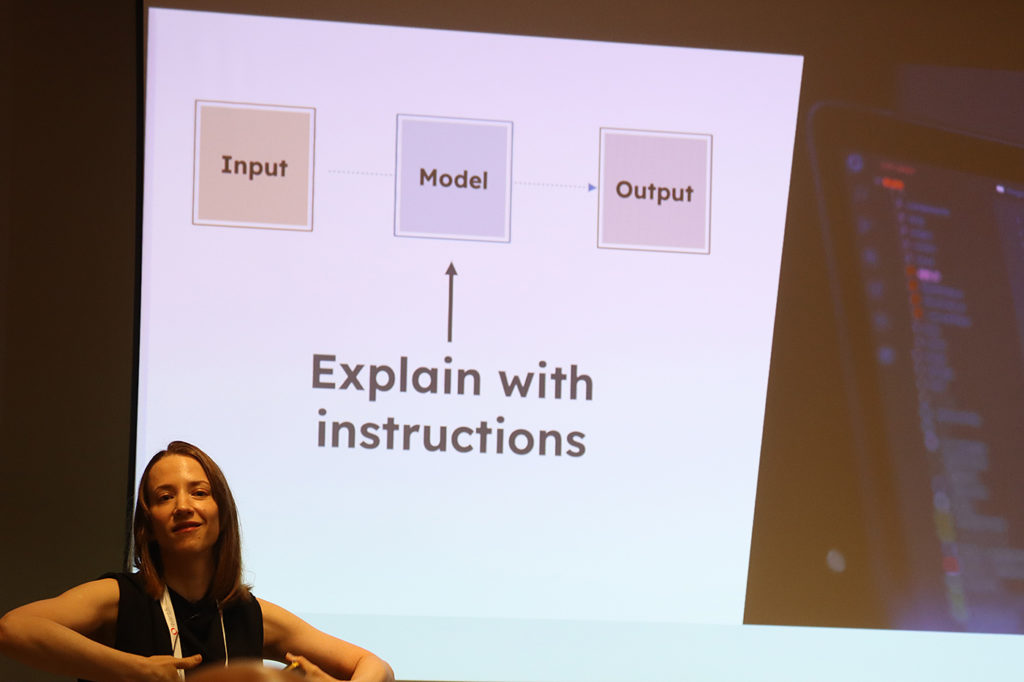
ADIA had large presence at the conference, with many speakers. In a relatively short space of time, it has built up a large quant team, with some of the most well known researchers joining the firm, amongst them Marcos Lopez de Prado, whose books on machine learning in finance have become amongst the most popular.
Lopez de Prado presented his take on the future of quantitative investing. He noted that financial markets are becoming ever more complex. He noted that in order to research them, having a siloed approach might be challenging. It was important to approach from an assembly line perspective, to enable cospecialisation. This way, you would have specialists at each part of the research stage, such as in the cleaning and preprocessing of the data, in fitting the models etc. One thing we have found at Turnleaf Analytics is the importance of every single stage of our pipeline for forecasting inflation with machine learning. The stages of cleaning and preprocessing the data are crucial. Hence, having expertise at each part of the pipeline is key to extracting the most value.
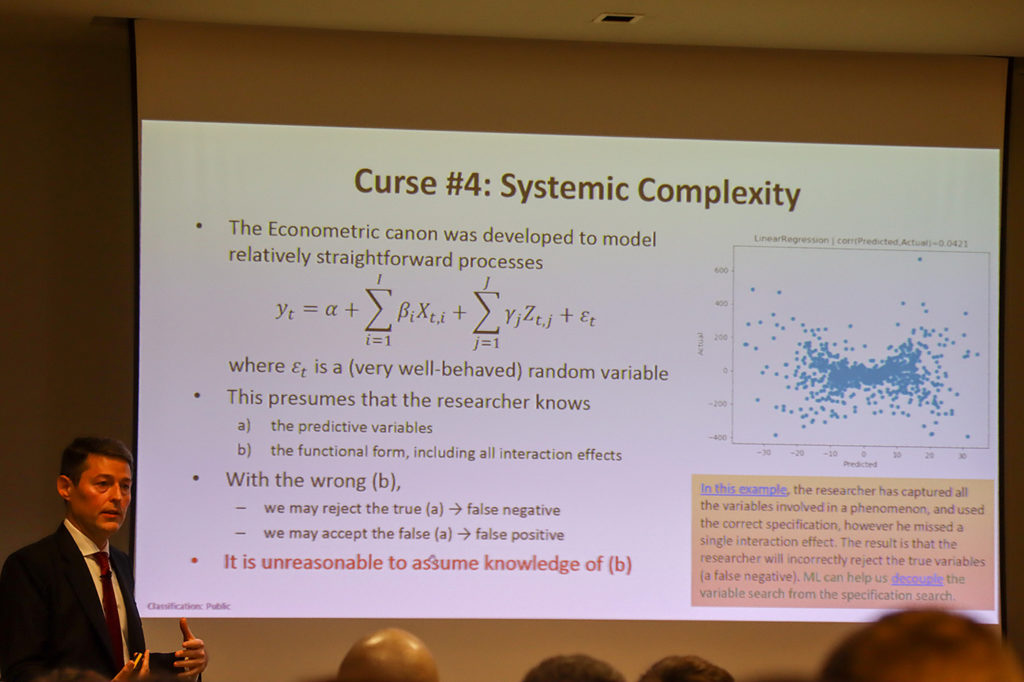
Rama Cont discussed the concept of excursions away from a reference level to understand dynamic trading strategies, effectively cutting up paths of price action into excursions. This contrasts to looking at the price action at regular intervals.
One of the things that is obvious for anyone working within quant finance is the small number of women working within the field compared to men in most organisations. QuantMinds organised a panel discussing women in quant finance, chaired by Katia Babbar, with panellists Roza Galeeva, Diana Riberio, Mirela Predesci, Svetlana Borovkova and Leila Korbosli. The audience also took part in an extended Q&A. Amongst the many topics raised at the event, they included the observation that other industries, such as consulting tend to have more female representation than banking, in particular in the quant arena. It was also noted how a similar skew in representation is visible at university in subjects like quantitative finance.
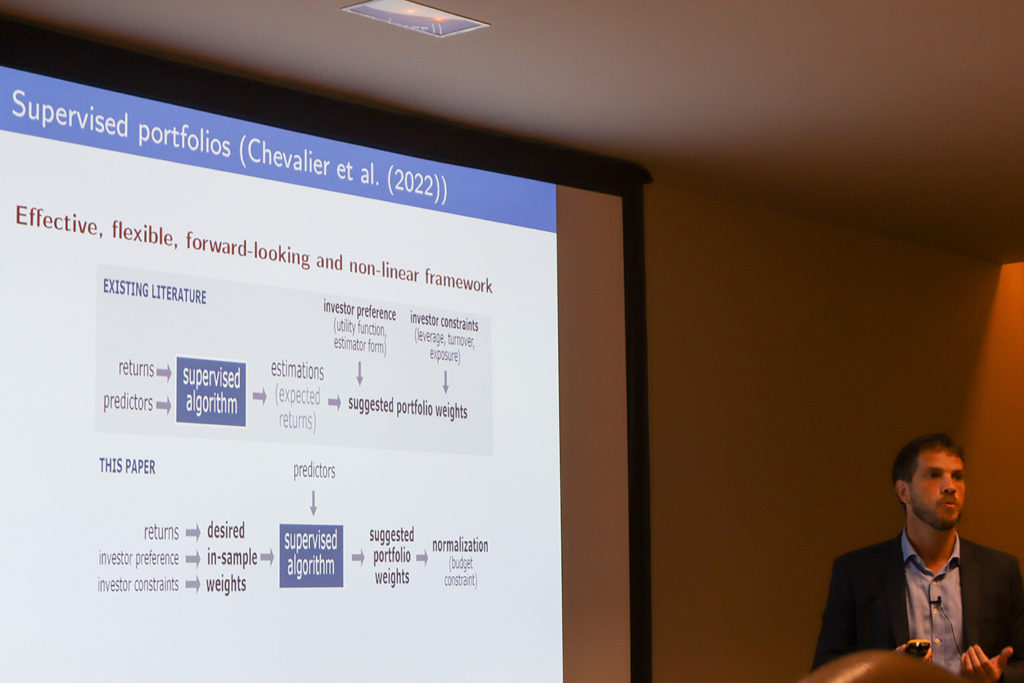
Thomas Raffinot (AXA) give a presentation on interpretable supervised portfolios. The idea was to forecast expected weights in the portfolio, rather than expected returns. One of the issues with any machine learning approach was that of interpretability. Raffinot noted how there wasn’t any real consensus about interpretability in ML. He discussed some approaches such as SHAP and LIME to explain models, or the alternative of using an inheritantly interpretable model, such as decision trees. He later discussed the use of RuleFit which could derive rules and also using a linear regression, essentially a simplification of a model, using it on a dataset of Boston housing and later in the context of constructing portfolios.
In recent years, discussion on crypto markets have become more frequent at QuantMinds. This year, Carol Alexander gave a presentation on NFTs, explaining how their rarity could impact their value. In a sense owning a particular NFT could be akin to being part of a club, and could be one plausible explanation as to why they have (at least in the past) attracted high prices.
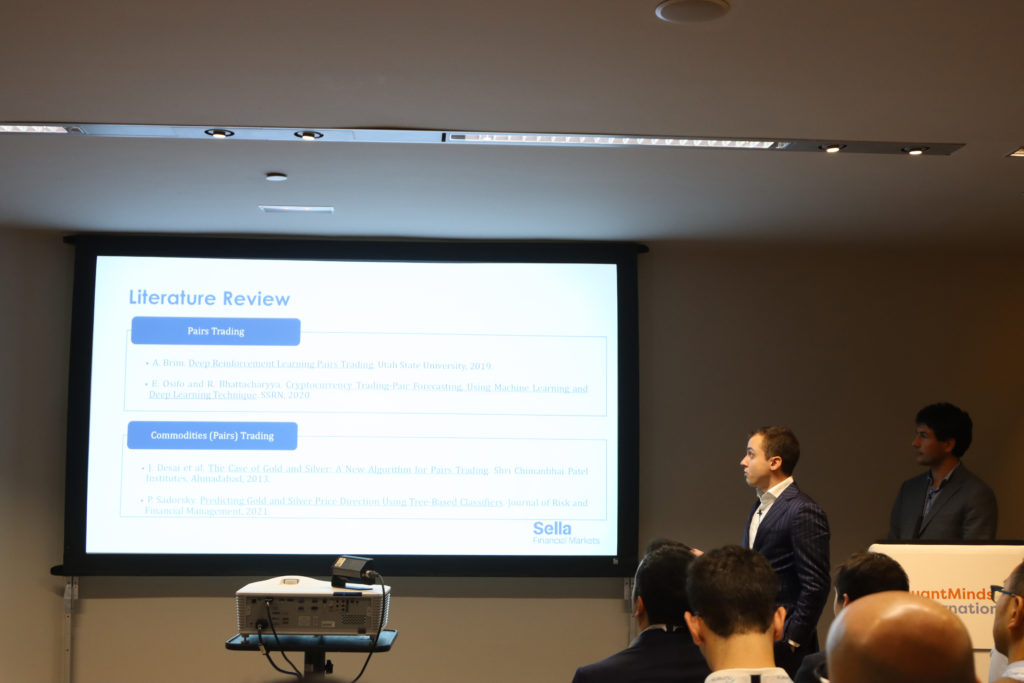
Matteo Rolle and Gabriele Biasin presented a machine learning approach for doing pairs trading. The data was high frequency market data. They tried a number of different models, SVM, random forests, neural nets and LSTM. The model they developed had a good enough accuracy, that it was also being run live with real capital.
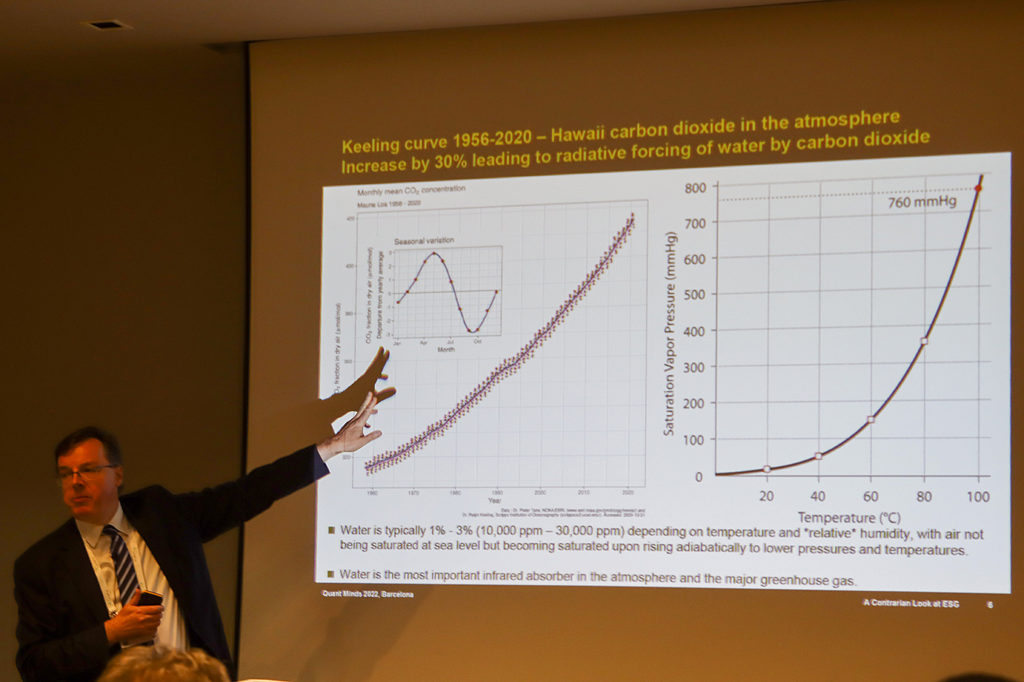
Erik Vynckier took a look at ESG from a more contrarian perspective, discussing the climate change within the context of historical changes of temperature, which have been a lot larger. Whilst, I didn’t necessarily agree with some of discussion, it was interesting to hear a different perspective.
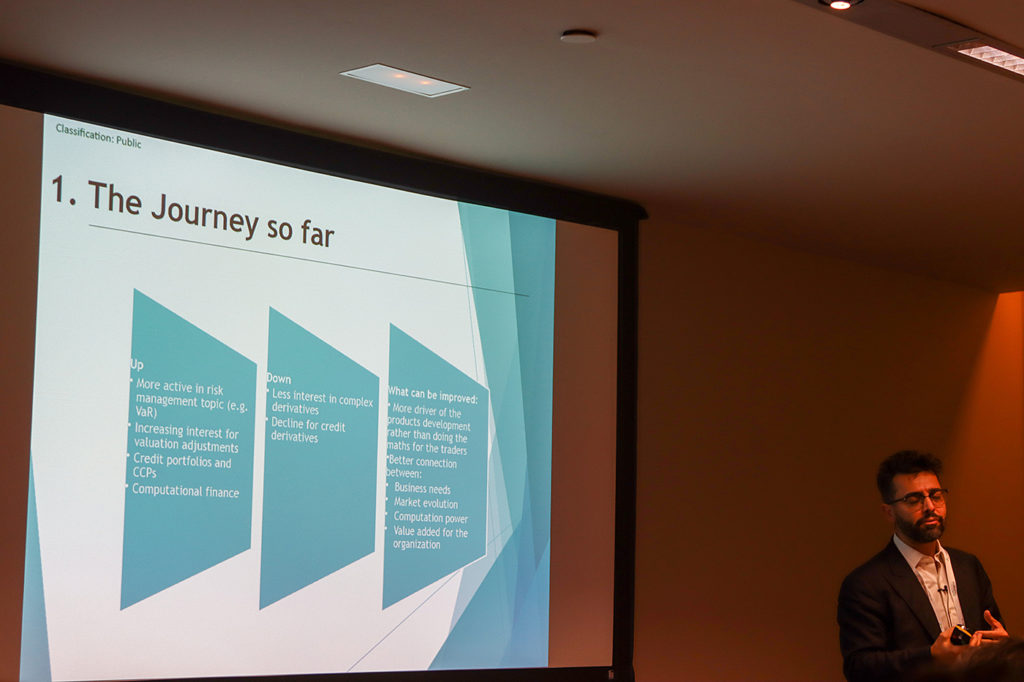
Maurizio Carro discussed how the quant world had changed over the years. In recent years, he noted how there was more of an active interest in risk. However, there was less interest in pricing complex derivatives. What were the challenges for quants? First, developers are quants. Second, it was important to unlock the promise of alternative data. Our experience in forecasting inflation is that of course how you construct a model is important. However, the data which the model ingests is incredibly important. Having the right data as input can improve accuracy significantly. Also quants needed to be part of the process not just for creating models (which you would expect), but also in monitoring subsequent performance of the models they create.
In general, the challenges more broadly were numerous, such as the use of ESG in models. Users also needed to understand the weakness of models, and what they can’t do. Having a knowledge of the products they are modelling. There was also an increased focus on quants to understand trading strategies. Quants these days are just as likely to be encountered in alpha generation roles as they are in option pricing.
In conclusion, QuantMinds definitely provided a lot for me to think about the quant field. It was great to catch up with my fellow quants in person, something which has largely been difficult in recent years during the pandemic. The trend towards machine learning is getting a lot more traction as evidenced by my discussions at QuantMinds, and I expect that in future years we’ll see this trend continue. I’m very much looking forward to next year’s QuantMinds event, which will be held in London.