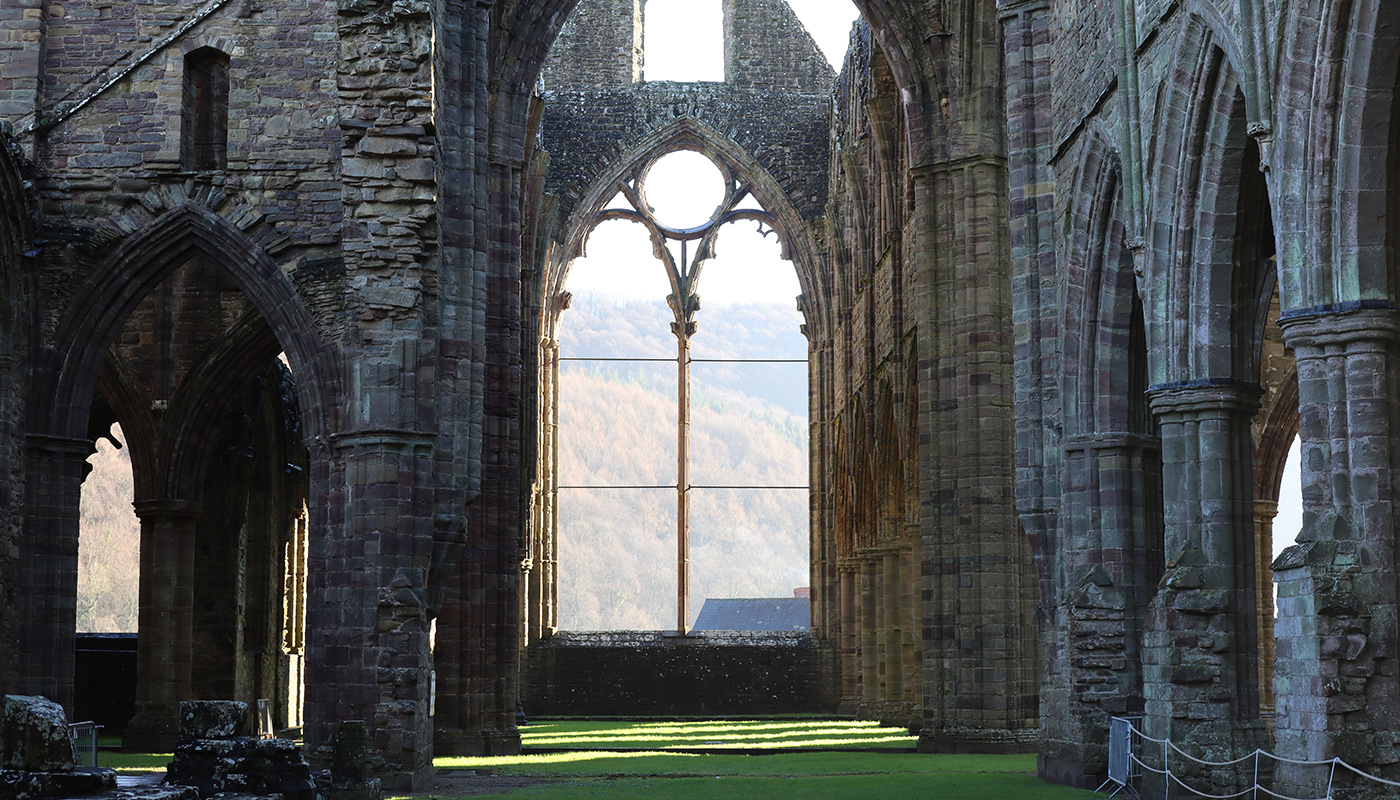
When visiting a new place, there are a number of ways of deciding where to eat. One way would be to check a website like trip advisor. However, you might feel that this isn’t the best way to research this. Perhaps a more authentic way to find a nice place to eat is to simply follow the locals and see where they dire. Where do the locals queue outside, as opposed to where the tourists congregate.
Does this work as a strategy? At least in my experience, it’s going to be one of the best ways! However, it can still be hit and miss. How many times have you queued outside a restaurant for a long time only to find it really isn’t anything special. But the key point is that me observe what others are doing before making our decision. In financial markets, we of course want to do our own analysis to come with our trading ideas. However, part of the process inevitably includes observing what others are saying and even more importantly what they are trading. As quants, how can we model what others are doing?
There is a lot of data out there, that gives us a partial look to understand what others are trading. Clearly, one of the most obvious datasets for understand what other people are doing is observing the price (cue, the comments of more buyers than sellers etc.!). However, aside from the price can we use?
Another common dataset, is that released by the CFTC which details positioning in futures markets split across different groups like non-commercial and commercial accounts. Typically, one use of positioning data is to identify extremes, and hence washouts. The difficulty is that it can be incredibly challenging to identify points of washouts, and in practice, when I’ve looked at it in the past, it more often than not pays to go with extreme positioning. Basically, extreme positioning is not in the only precondition for a washout.
The CFTC is only released once a week and the data is also lagged. Some folks basically ignore this data as a result, but I’ve found some value in it in the past, with a bit of work. It is also possible to find other datasets which offer much more up to date data for certain markets like CLS, which has a very detailed FX flow dataset updated at high frequencies, which I’ve researched. The more OTC an asset class, the more difficult it will be to gauge flows and market positioning, if you do not directly observe this yourself (eg. a large market maker). Each asset class will obviously have different datasets for flow/positioning. More broadly we can get flow datasets which describe many other aspects of the market, such as tracking cross border fund flows etc.
We can also get proxies for market sentiment. We can look at extracting sentiment from machine readable datasets or other datasets such as social media. We are likely to get different signals from newswires versus social media, so combining them conceptually should give us different information. We can try to combine positioning data with market sentiment. If folks are heavily positioned long, then we might expect bad news to have more of an impact, then if everyone is already short for example.
Of course assessing fundamentals are important for any trader, and if you are trading systematically, some factors based on fundamentals are useful (eg. for FX, modelling economic fundamentals), however, understanding what other market participants are doing is important, because we are not trading in a vacuum. The market reacts not only to your trading but everyone else’s, hence modelling factors like positioning and sentiment are useful too.