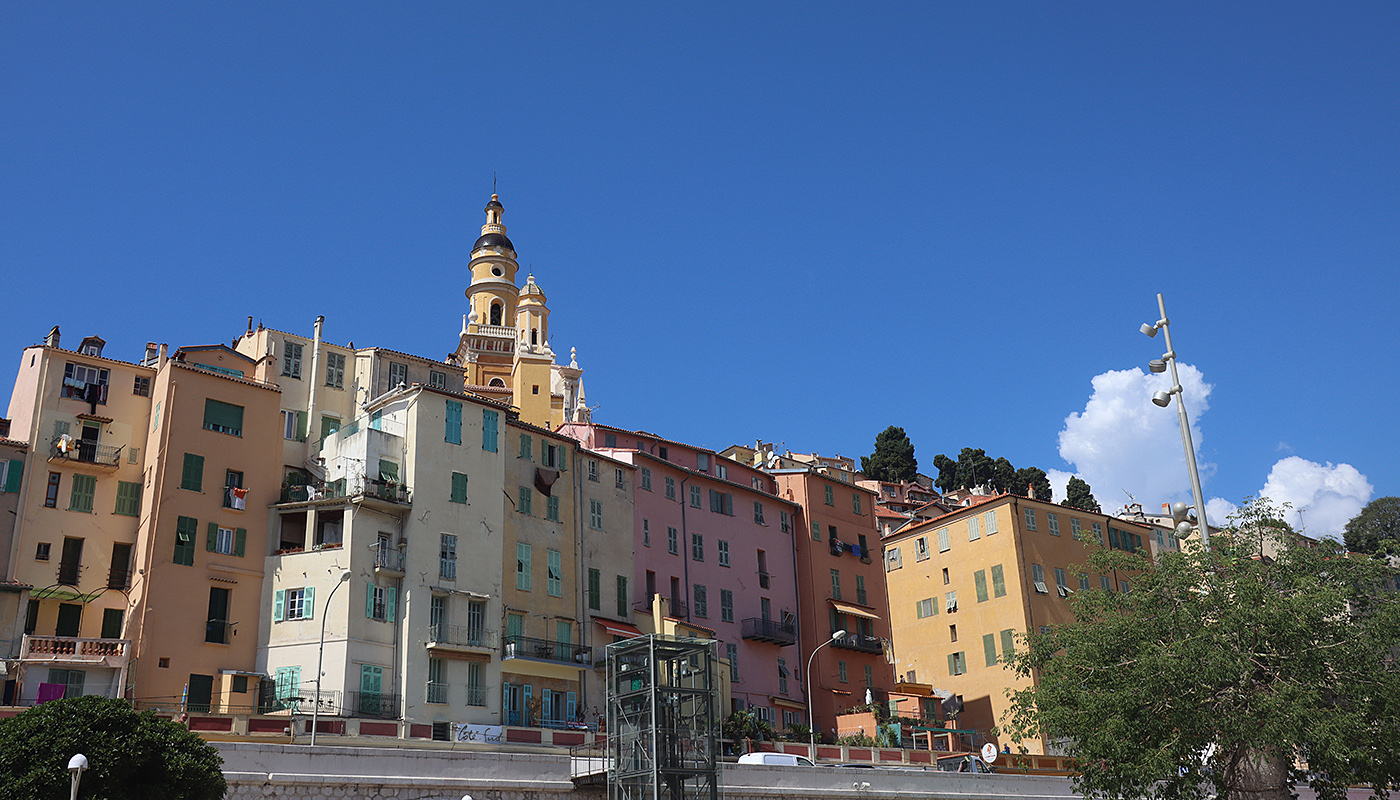
In big cities, there is always a flavour of the month. A new restaurant opens, and amid a lot of buzz, it’s impossible to get a table. After a while, the fuss dies down, and it’s suddenly a lot easier to get a table. Then there are other restaurants, which aren’t particularly fashionable, but serve good quality food consistently. I’ve got to admit, it’s probably to the latter places that I tend to go to.
In financial markets, the concept of flavour of the month is also common. This month, the theme that everyone is (unsurprisingly) following is that of inflation and is driving markets. You really don’t need to be scouring CPI data to notice that prices seem to have gone up recently (and what you get has shrunk, including the portions in restaurants). However, data is nevertheless a valuable commodity, to understand where that inflation is concentrated and further more to help us forecast whether or not inflation is “transitory” or not.
If we are a discretionary trader, we might therefore think that the datasets we use in our analysis, should also be thematic, this month inflation, the next month politics etc. By contrast a systematic trader, will try to identify datasets which they will use persistently over time, rather than using their discretion to flip in and out various models repeatedly.
I would argue that the way a discretionary trader should utilise datasets should actually be closer to the way systematic traders use datasets, in a more persistent manner. Of course, there will be periods where certain themes are going to occupy markets and there should be a focus on them for research by discretionary traders. The difficulty is that it takes time onboard datasets from an operational perspective, whether it’s signing contrasts, negotiating etc. (although for more common datasets on aggregators this time will be reduced). However, no matter how you purchase a particular dataset, there’s also additional time necessary to parse, clean, research, model and understand any particular dataset.
Hence, if we wait till the last minute before subscribing to a dataset in order to investigate a current market theme, we’ll be faced with a large delay, before we can get an answer. By contrast, if we have already subscribed to a particular dataset, which we have already researched and modelled, and are continually monitoring signals, we’ll be able to act quicker and any signals might be generated earlier. The ideal case, is that we have many models built on a multitude of datasets that continually monitor markets, which can flag to discretionary traders if there’s a particular factor that needs research.
It’s understandable that we only want to subscribe to datasets when they are most relevant. However, this approach means that we will be slow to extract the answers we want from a particular dataset, at the time we need it most. It isn’t only systematic traders who should think of datasets as persistent resources, but also discretionary traders.