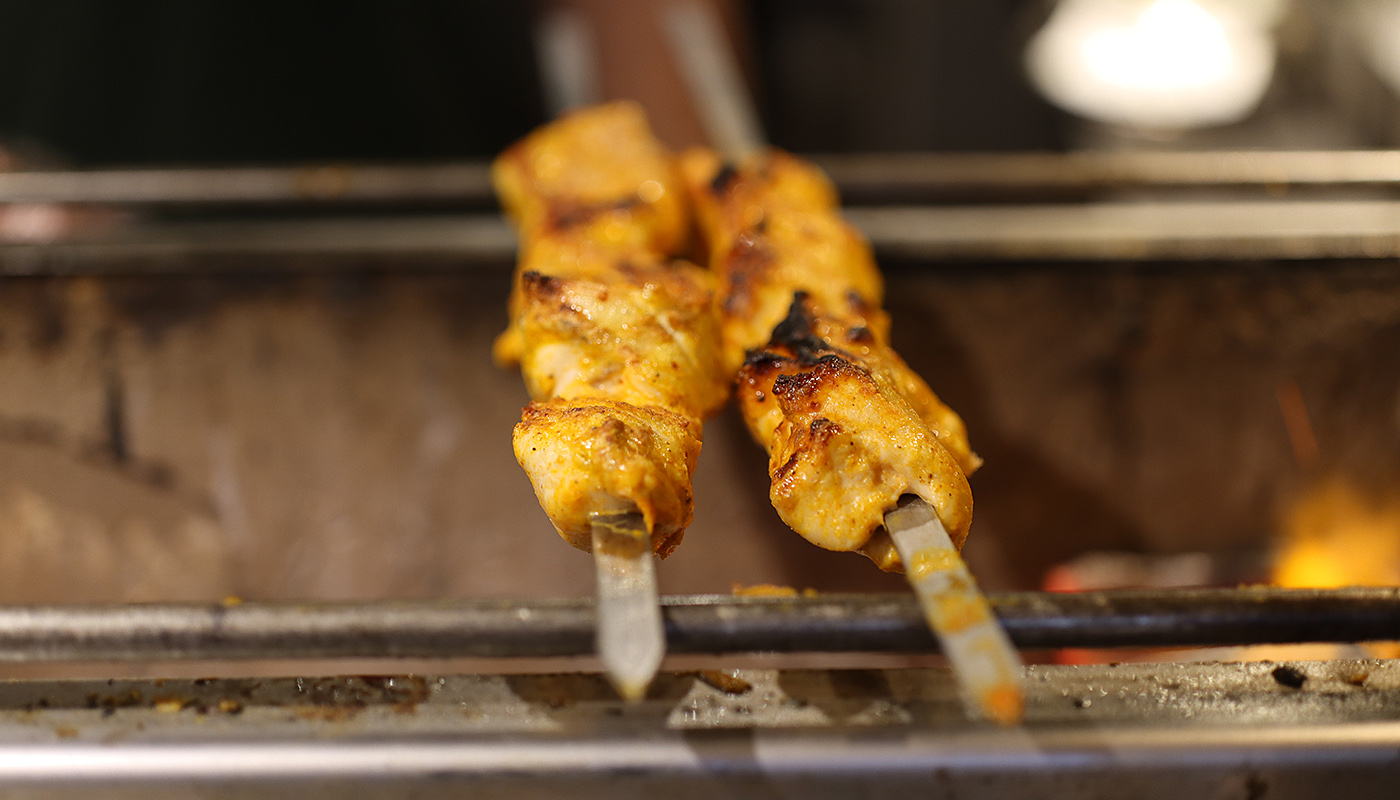
If you’re building a house, what’s important? You need an architect to design the house alongside civil engineers. You need builders to, well, build the house. Of course, you could try your hand at some of these tasks, but it’s going to be a very steep learning curve. It’s also one thing trying your hand with a bit of DIY, and quite another to build a house. I’m writing this from the vantage point of someone who is truly useless at DIY. For years I could stare up at my ceiling and see what a mess I made of painting my own ceiling (this is what an estate agent would call character in a property).
Suffice to say, next time it came for refresh of my living room, a professional painter was employed. We also need to spend a considerable amount of money on building materials, as well as a plot of land and we need to navigate planning permission too. In short the whole process is non-trivial, but this is essentially a very simplified summary of what a developer does.
We can reuse this analogy to think about how investors make their trading decisions in a quantitative environment. We can think of the building materials as data, the raw input into our decision making process. The architects and engineers design the framework for how this data is used, developing trading models and strategies. The builders implement this framework in code. The final client for all this building work is a portfolio manager, who uses as part of their decision making process. The difference with a real “house”, is that the portfolio manager should be involved in the whole process like a loop continually talking to the “builders” and “engineers”, so that any outputs in the process are relevant. A client wants a house to built to their specifications, not to what the builder who wants. When it’s the other way round, you’ll end up in endless disagreement, with neither side happy.
When traders want to incorporate a quantitative input to their decision making process, it can be tempting to look at only one aspect of the building work. The difficulty is the process doesn’t really work unless its done as a whole. I’m not suggesting hiring a massive data science team from day one to start with, but more about considering what resources are needed. If you skimp on “building materials”, ie. data, then your data scientists will have nothing to build and sitting around. No data, no insights! Similarly, if you lavish spending on datasets, but don’t have sufficiently qualified folks to do the number crunching, build models and interpret them, you are not to going extract sufficient value from your data. If there is a lack of communication between all the parties whatever gets built won’t be usable, by the portfolio manager, and a waste of resources, both in terms of time and money. A model is only going to be of value if the insights you glean from it will be used in decision making.
There are many hurdles to properly incorporating data and quantitative tools into the investment process. Furthermore, the precise way it needs to be done is different for a quant fund compared to a discretionary fund. The key is to make sure that work is done to make sure that we have multiple parts of the puzzle, whether it’s a budget for data, having the sufficient skills to number crunch it etc. We can’t have only piece of the jigsaw, and wonder why it isn’t having an impact on our investing.