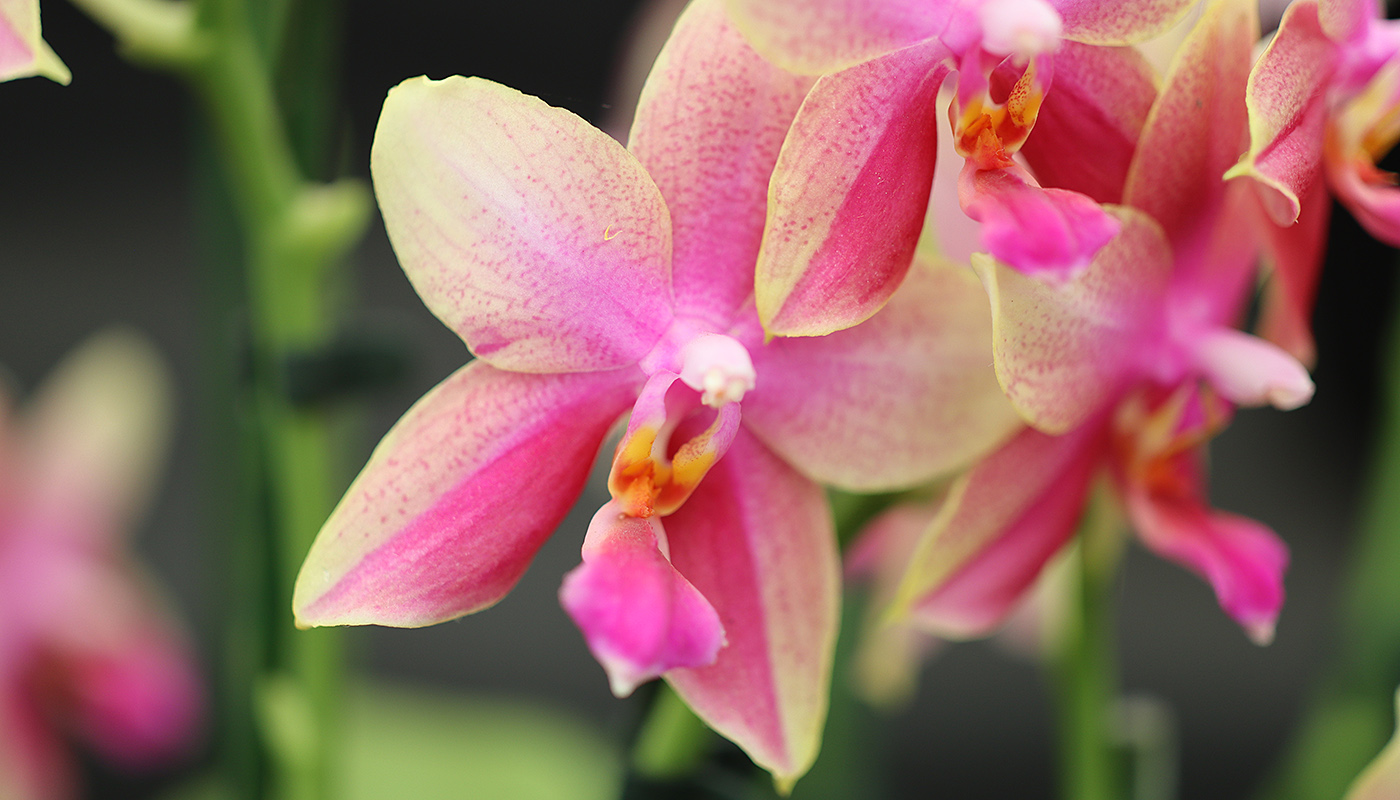
Every year that passes, it seems to become clearer that there is even more I don’t know, in particular those areas where I’ve worked the most, financial markets. If it’s a new area, then it’s a lot easier to assume you know than you know, because you are shielded from a lot of the complexities. Somewhat perversely someone who is an expert in the area, is probably more aware of the unknowns and difficulties associated with that area.
I’d argue that this is the case in the financial markets. To an onlooker, it might seem “easy” to make a lot money. All you had to do, was to be long bitcoin a few years etc. However, for anyone who has worked in markets for many years, it seems a lot clearer, that in practice being consistently profitable is a challenge (or at least to outperform a benchmark such as S&P 500). Whenever, we create a trading strategy or a forecast for a particular market or economic variable, we have incomplete information and many unknowns.
How can we fill in our blind spots, when it comes to financial markets? The most obvious unknown is data from the future! There’s not much we can do to change that. However, what we can do is to have as complete a view of the market in its present state as possible. That means trying to seek out as much data as we can to describe the market. Alternative data can help us fill those gaps.
There are several ways we can describe these gaps. Some of these gaps are related to timeliness. Alt data can give us insights quicker than traditional data. Take for example credit card transaction data. Once aggregates it gives us up to date insights on retail sales much quicker than official data. The second aspect of alt data is that it can tell you something you didn’t know. For example alternative datasets within FX, can give you an idea of what FX flows look like, which historically has been far less visible, and often only information that large market makers would have.
Sometimes just using one alternative dataset, won’t appear to get you any sort of signal. I’d argue that one of the most difficult things with alt data is that often we need to combine together many different datasets to get the best signals. It also takes a lot of thought and imagination to think about use cases for many alternative datasets too, and to structure those datasets into a usable form. It’s often the data preparation and cleaning that is the most time consuming stage of the analytic process. There’s a lot we don’t know about markets, most notably the future! However, alternative data can help plug at least some of those gaps in our understanding.