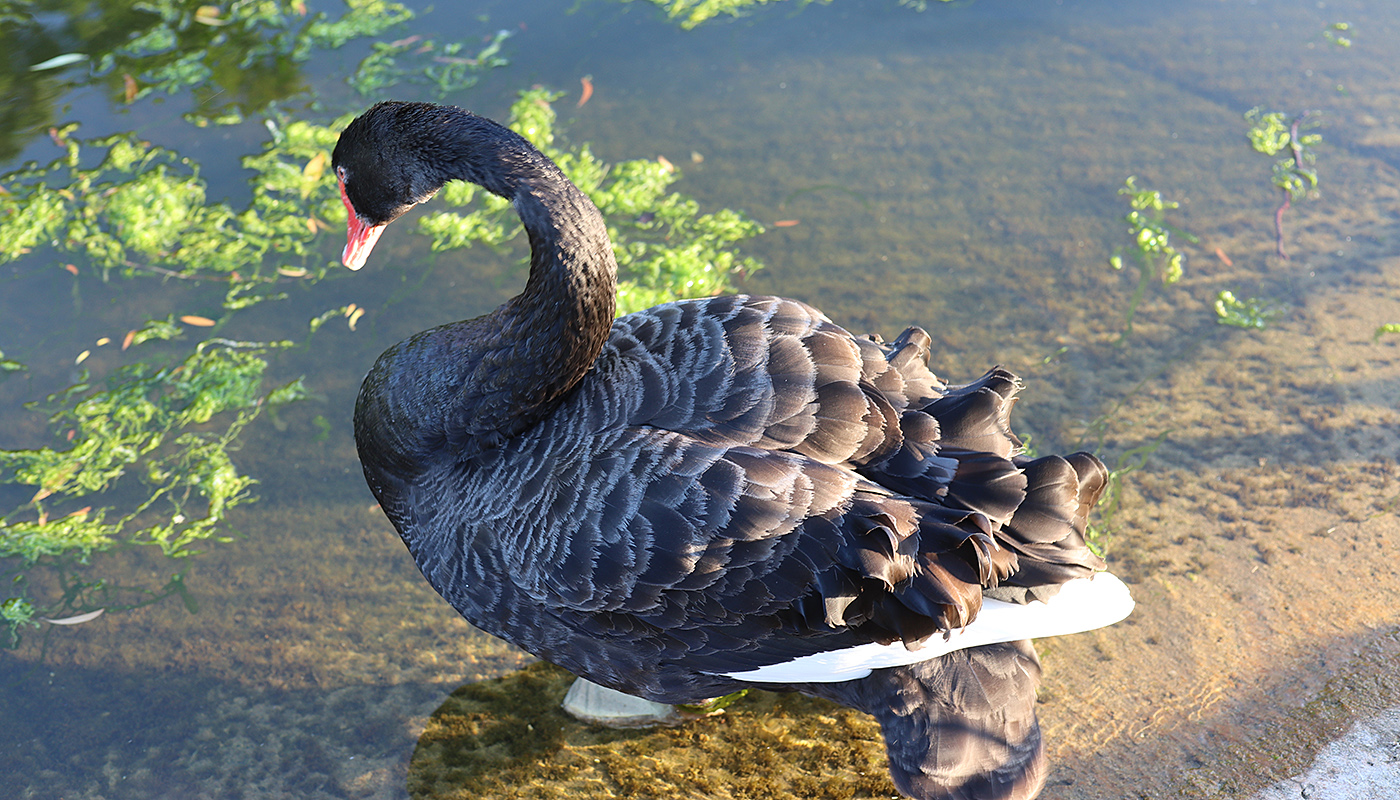
We all have budgets in mind for certain things. If someone offered me a burger for 1000 GBP, well, I’d probably have to politely decline. If someone offers me a house for 1000 GBP, well, that seems like good value! Our budgets are related to what we perceive as being “good value”. Ok, that’s fairly obvious.
When it comes to alternative data, one thing of the most difficult problems is trying to value a dataset and it is something that Alexander Denev and I discussed at length in The Book of Alternative Data. How you value a dataset will depend upon your perspective. The data vendor will have one way of valuing it, namely the cost of production and the margin they might be able to charge on top of that. The user’s perspective will differ depending on whether they are a discretionary trader or a systematic trader.
For a systematic trader, they will try to quantify the value of a dataset, by assessing how much additional alpha it can offer versus existing models. If a dataset is very profitable, but seems to deliver no additional alpha compared to their existing strategies, it will have no value. Another systematic trader might come up with a different value, simply because their existing strategies are different. For a discretionary trader, the dataset will also have value if it can tell them something they didn’t know, or help to corroborate other datasets, but of course it is more difficult to “quantify” this in this context.
However, the budget for alternative data isn’t purely the cost of purchasing the data itself. Even if we restrict ourselves to free datasets, we need to have a budget! There needs to be a budget for the use of a tech stack whether it is for local servers or the cloud. We also need a budget for data scientists, data strategists and data engineers. Every single data trial might be “free”, but still needs to be supported by our data team, who will likely need to be put in quite a lot of time.
What if we restrict ourselves to “free” datasets, but this will inevitably reduce the types of alternative datasets that we can access. It can also the case that “free” datasets might actually end up being more expensive! In particular, we might find that we can indeed get access to “free” data, through tools such as webscraping. However, the cost of structuring and cleaning the data (and maintaining it) ourselves might end up being significant and outweighing paid datasets. A data vendor has economies of scale, because they are providing datasets for many clients, hence they can spread the cost of cleaning and structuring a dataset amongst them all.
Alternative data is an important tool to shed new insights, but if we want to use it extensively in our process we need to allocate budget for it, not just for the data itself but also for the resources to deal with it. If we are not prepared to allocate any resources to alternative data, we are going to have difficulty extracting any value from it.