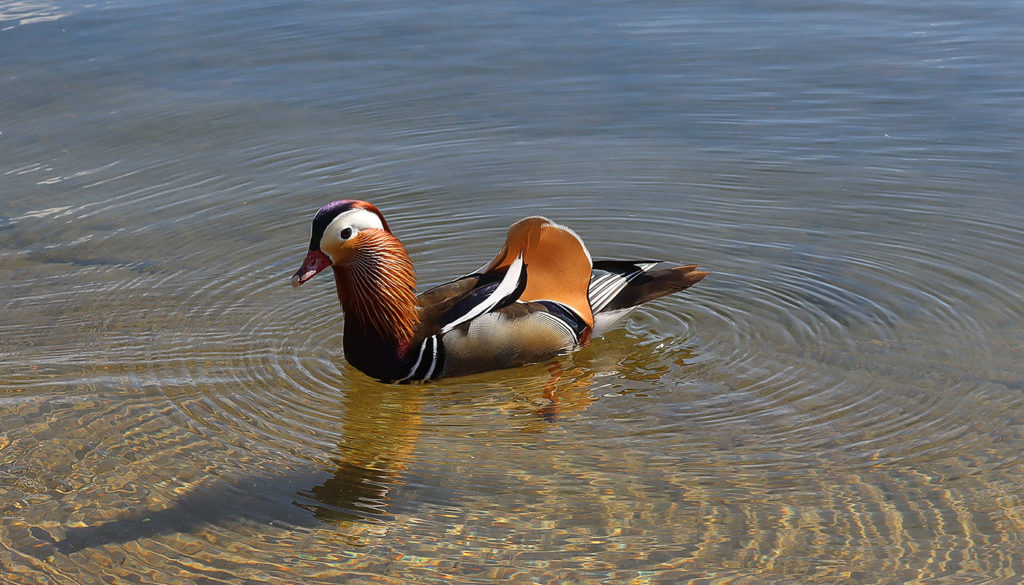
Let’s say you want a burger. How do you find a good burger joint? One way is to try all the ones nearby, and then when you done that, pick the best to return to (this is the research stage..!). In practice, this isn’t feasible, there are just too many! Instead, the only way to do it efficiently is to create a shortlist of potential burger joints and then select from that. You might use reviews to make up your list, recommendations from friends, as well as your own knowledge.
If we think about financial markets, whenever you want it analyze them, it often requires understanding what data is needed. Some of the datasets are relatively obviously, like market data. However, when it comes to alternative data, it’s a lot more challenging. There are many alternative datasets to choose from. Just like with our burger example, it isn’t feasible to test every single dataset to ascertain if it has a signal. We need to make a shortlist in order to help us understand which alternative dataset could be useful. This shortlisting process can often include talking to data vendor‘s and aggregators. From this shortlist we can pick the most relevant ones to test.
In The Book of Alternative Data which Alexander Denev and I cowrote, we wrote about various criteria which can be used to create the shortlist. Firstly this would include understanding whether these datasets are relevant for the assets we wish to trade. Let’s say we have a dataset which helps us identify how many Apple iPhones have been sold. This might be relevant for trading Apple stock, but if we are trading EURUSD it is likely to be less relevant for us. Also we need to understand the frequency of the dataset as well. Again this is an easy to use metric to identify whether it’s relevant for our use case.
We also need to understand the cost of the dataset as well. If the dataset is very expensive we would need to demonstrate a significant improvement on our signal in order to justify purchasing it. Understanding the added value of a dataset can be particularly difficult if we are discretionary traders. For systematic traders however it should be somewhat easier because we can identify how much value it might offer compared to how are existing data sets in a systematic trading strategy. However even for a systematic trader it is not necessarily the case that we will be able to extract the signal. This can be either because there is no signal or because our analysis has not been sufficiently thorough to identify signal.
At its most basic the research process might involve doing correlations between our alternative dataset and the asset that we wish to trade, but later on they can be much more involved in terms of constructing signals and in particular because we often want to combine many data sets together to create a signal. We can’t have the situation where a dataset in isolation might have a weak signal but in combination with others provides a stronger signal.
The above criteria we’ve mentioned for identifying whether to test a dataset is not exhaustive, and we mention many more in the book (and I’m sure there are many more criteria we could have included). However having some way of shortlisting data sets is key to using alternative data. This is because the evaluation and research process is very time-consuming, so having an efficient process for shortlisting can add a lot of value to your firm.