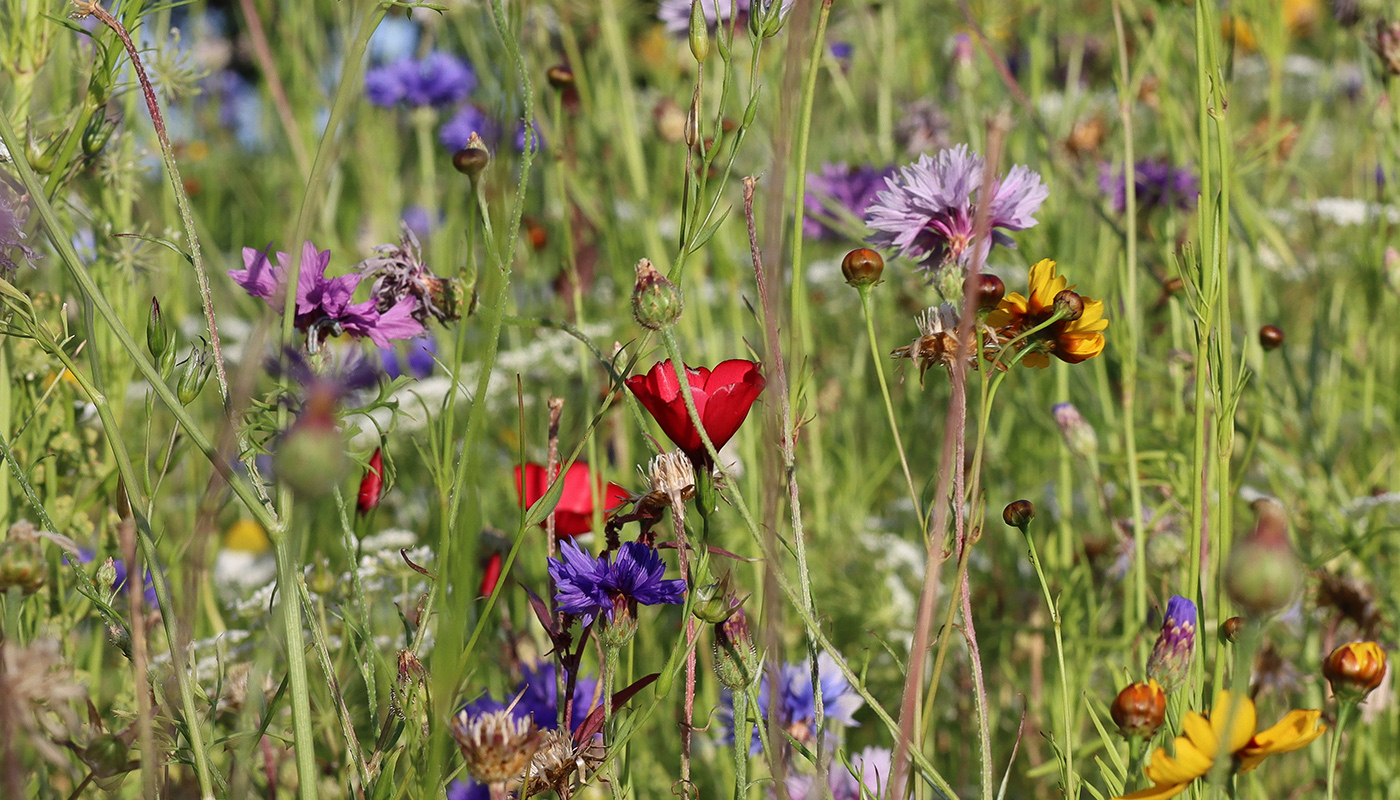
If you live in the UK, the weather is always a topic of discussion. Sometimes it rains (well, a lot of the time it rains), other times it’s sunny. What’s somewhat perplexing is the sudden change within a couple of hours. Admittedly this isn’t something unique to the UK, many countries have changeable weather. The only way to work out whether to take an umbrella is to look at the weather forecast. Typically, on the TV forecast, they’ll say it’s raining or sunny etc. for the whole day.
However, in practice, is that forecast sufficient to make an informed decision, whether or not you need to take an umbrella? In practice, we can make a better decision if we have more granularity around the forecast. For example, can we get a more detailed forecast broken down by hour of the day, rather than for the whole day? Can we also get some probabilities around the forecast? If the chance of rain is say 5%, I’m willing to try my luck without an umbrella. If it’s 25% maybe I might take a small umbrella as a hedge. If it reaches 50%, then I’ll take the big umbrella! Suddenly with more granularity around the forecast, we can make a more informed decision about how we interpret it.
With financial markets, getting granularity around precisely what a forecast means, is just as important. We might see that the market consensus for a particular event is a certain number (let’s say for nonfarm payrolls, the outcome of a Fed meeting, likely move in an asset following an event etc.). However, just as important is understanding how uncertain that forecast is. If the distribution of forecasts for a data number shows a massive difference between the high and the low estimates, maybe we shouldn’t attribute quite so much weight to a consensus figure. Furthermore, what if the consensus number might also mask precisely how the skew of the underlying inputs? The distribution of the forecasts is just as important as the actual median number. This is obviously just as important for events like the US presidential election happening shortly, understanding the relative probability of the outcomes.
Our umbrella example also helps us understand how we manage risk around these events. Even our portfolio is clustered around the consensus view, if there is sufficient probability of a negative event occurring, then it might force us to think about precisely how much risk we’ve allocated or if we need to have a hedge. Ultimately, the future is only one possible path from many. We need to be sure, that if we get the “wrong” path, it won’t be disastrous for our portfolio and furthermore, we need to think about the probability of those wrong paths occurring.