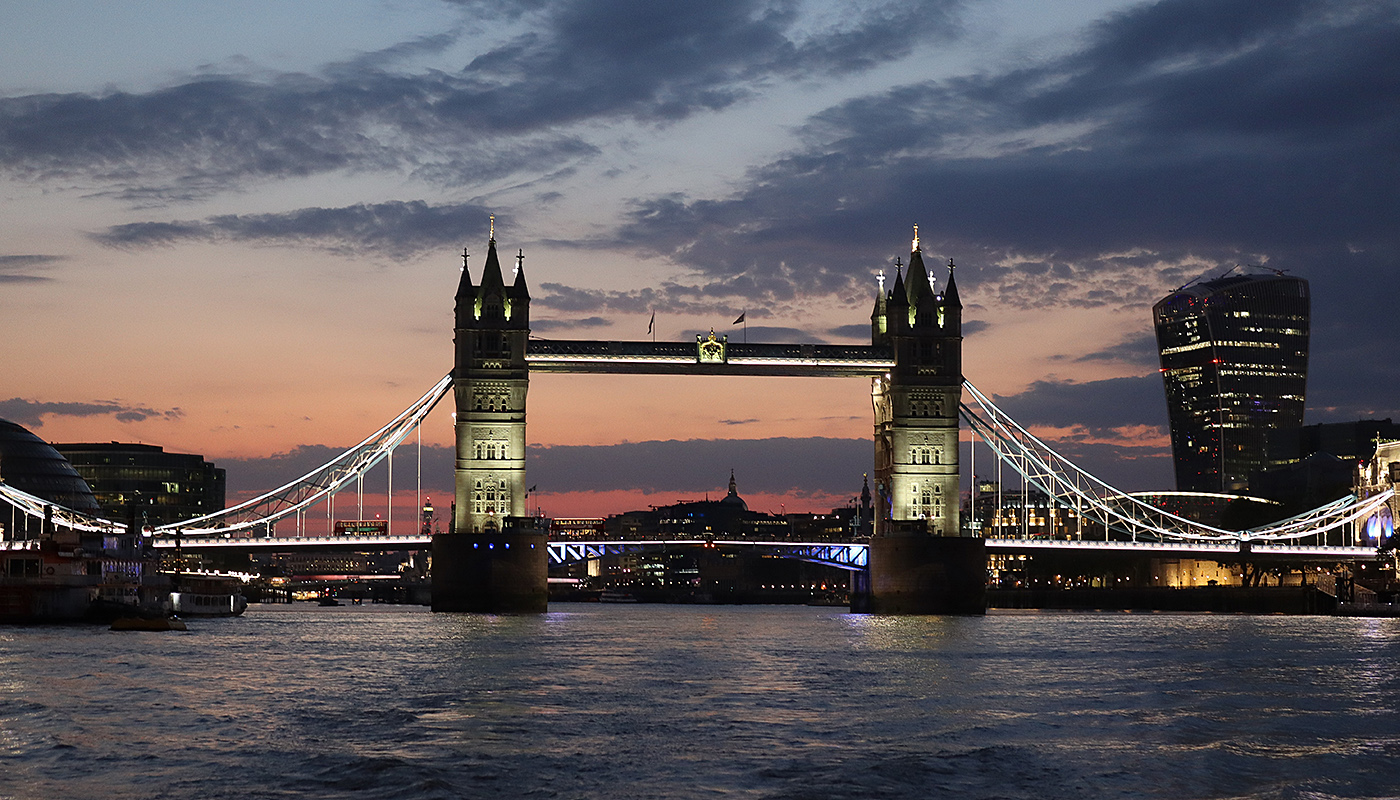
Most times I go to a burger joint, I seem to be happy. Is there correlation or causation, when it comes to my state in these instances? I would argue that there is some causation going on here, in that going to burger joint makes me happy. I’m pretty sure, most folks would agree with this.
In financial markets the whole notion of causation and correlation is much more muddied, and it can be tricky to disentangle the two. One such area is technical analysis. Are markets reacting because lots of market participants are looking at the same technicals and chart patterns and their behaviour in markets is causing a self fulfiling loop, because stops are clustered around these levels? Or is technical analysis simply some tool for explaining what has happened and it’s just a coincidence, which doesn’t have a predictive power?
I recently had a Twitter exchange about technical analysis and whether or not it works. I’ve also been reading The Art of Currency Trading, by Brent Donnelly, who I used to work with at Nomura. It’s a great book, and one of its sections discusses the topic of whether technical analysis works. You’ll have to read the book yourself to get the full details, but the general gist of Brent’s view is that technicals can be useful, as part of the decision making process, although he’s more sceptical of the idea of purely successful technical traders.
I do know some exceptionally good technical analysts, who have been able to call the market very well, such as Tom Pelc, who was also a colleague at Nomura (and I recommend following him on LinkedIn!). That being said, it isn’t very easy to do technicals well, and I think part of it is that it can be very difficult to be disciplined when reading charts. If you have a view and simply search for technicals to fit that view, it kind of defeats the point (it’s the equivalent of data mining). Some technicals are also quite rules based, such as moving average crossovers, and it is possible to create systematic trading rules and test their historical profitability. It’s also possible to test the lack of profitability in others). If a chart pattern never works in a backtest, it is still valuable information to know to avoid using it (and to stick to those which are profitable). Others, however, like Elliot Wave are highly subjective, and can be very difficult to interpret and would be incredibly difficult to backtest.
You could argue that many systematic trend following strategies are essentially, very heavily disciplined technical analysis strategies (I’m sure not everyone will agree with this!), where absolutely everything is rule based, and you also have many overlays to calculate how to allocate risk across different assets (eg. with vol scaling). There’s also a lot of research which goes into managing execution too when running these strategies with large notionals. For the most part, these strategies have largely been profitable for decades, even if in recent years they have struggled a bit.
All these approaches, whether technical analysis or systematic trend following strategies are pure price based strategies. We might also create strategies which augment the price data of the asset we are trading with price data from other areas (eg. trading FX using interest rate data). Whilst price data is very important, there are lots of other datasets out there. If we ignore all other datasets we are missing out on information! This could include data on economic fundamentals and what central banks are doing. It’s difficult to ignore the impact of central banks, in particular recently (Cuemacro actually has a Fed sentiment index which does NLP on Fed communications too, which you can subscribe too!)
Just using price data also means missing out on the whole world of alternative data. This could range from credit card transaction data to give you a high frequency view on retail sales, or it could be news or social media text with sentiment tags etc. Alexander Denev and I have written extensively about using alternative data within your investment process in The Book of Alternative Data, which has been published recently.
Not every bit of information is necessarily reflected in the price, and this is even more likely to be the case for those datasets which are not commonly used be market participants and offer an orthogonal view on what’s going on. These additional viewpoints are exactly what alternative data can potentially give you. Of course, it isn’t easy, and it’s likely you’ll go through many datasets which don’t necessarily tell you much extra. Furthermore, different datasets will be relevant for different investors.
Technical analysis is an interesting tool, even if it’s possible to be sceptical about the area. But if we combine price based approaches (including technical analysis) with other datasets and signals, we’ll have a more rounded view of what’s going on in markets.