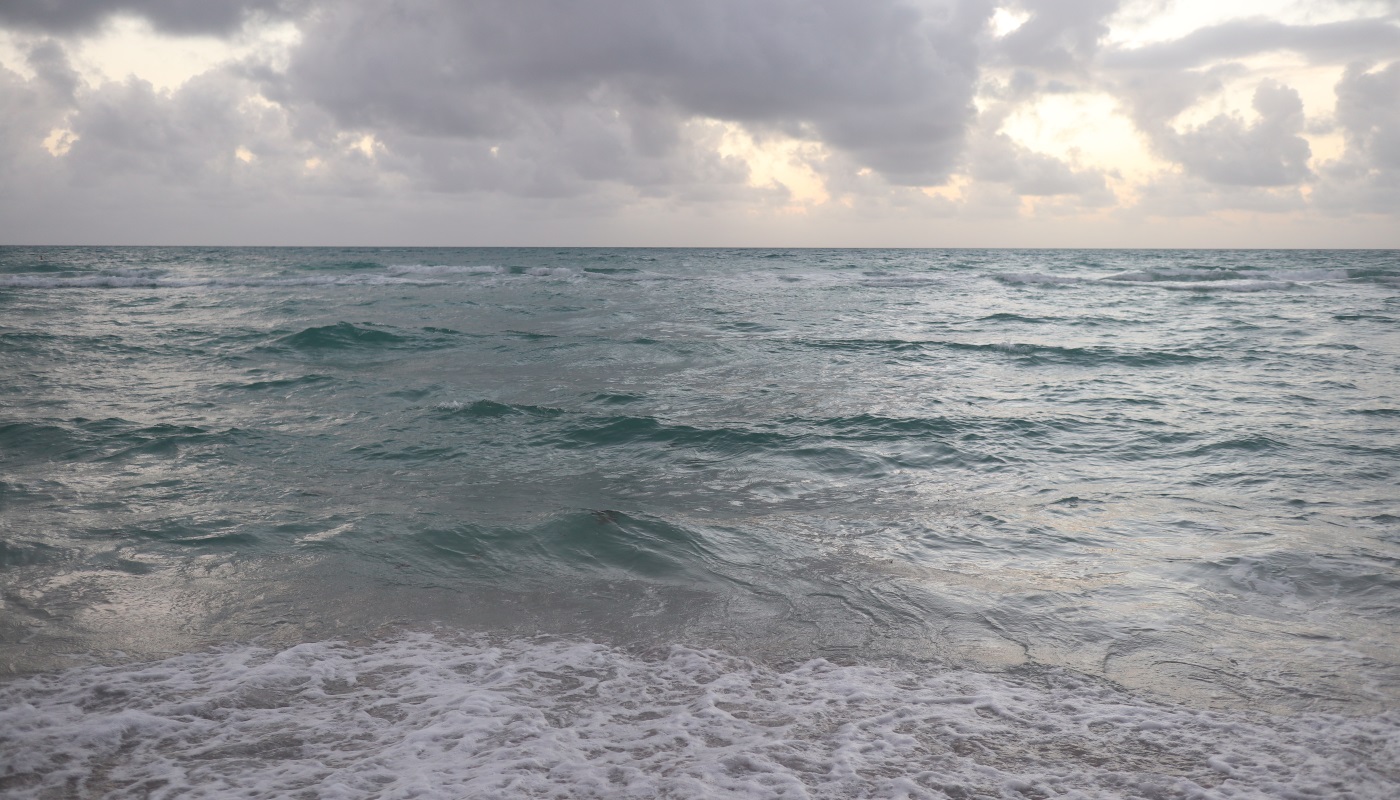
There are McDonalds everywhere. It’s probably the most ubiquitous fast food joint. If you want a burger, and want it quickly, it not a bad choice (double negative intended). Is it the best choice for a burger? My uncontroversial response would be, no. I doubt many readers would find an issue with my answer. There are many other burger joints which have much better burgers. It all comes at a cost however, not just financially but also in terms of time. You want a great burger, you’ll have to wait for it to be cooked, and it’ll cost more than a Big Mac. My point is that ubiquity and low cost aren’t always the only criteria you’d use to judge a burger.
Data, like burgers, is ubiquitous in our society. It drives the largest companies such as Google, and how you use data is not purely a question for tech firms. For investors, data has always been a key part of the investment process. Today there are more datasets available for investors. Some of course are traditional, such as market data and economic fundamental data. However, there had been a huge increase in alternative data, datasets which haven’t traditionally been used in finance. These can range from machine readable news to satellite imagery.
When it comes to buying data, what are investors looking for? If you want to know more about this, in The Book of Alternative Data, which Alexander Denev and I are coauthoring (available for preorder on Amazon), we seek to answer this question in a lot of detail. Here, we’ll try to summarise a few of the major points however.
Different traders want different things, when it comes to datasets
All traders want datasets which can help them make better predictions about markets. That is obvious. However, in practice, whether a dataset is useful depends upon the trading style of a trader. Just because a dataset sounds cool doesn’t mean it’ll have an alpha. There isn’t always a “universal” opinion when it comes to a particular dataset. I’ve talked to folks who have found absolutely no use for a particular dataset during the testing process, whilst others find it useful, even if they are the same sorts of investor. Often it can be challenging, because a dataset in isolation might not yield a strong signal, but it can be used together with other datasets to generate alpha.
You’ll have even bigger differences between say quant traders and discretionary traders. On the quant side, typically they’ll want a dataset that can be used for many names ie. broad dataset. In the discretionary market, folks tend to drill down into specific assets in a lot of detail, hence, are fine with only having data on very specific assets. There’ll also be differences depending on the relative frequency of their trading strategies. If you’re a long term investor receiving daily delayed data is fine (or even monthly), for high frequency traders, they’ll need to have much more frequent delivery.
Data buyers want clean data
No one likes cleaning data, and investors will appreciate it if a vendor can do this well. I’ve never heard a data scientist say they enjoy the process of cleaning data. Cleaning is, however, necessary to do all the fun analysis later. As the saying goes with data, garbage in and garbage out. For data vendors cleaning a dataset is crucial. There also needs to be some transparency in how the data has been cleaned.
An understanding of where the data is from
In order for an investor to use any dataset, they need to be sure that the data is sourced in a properly licenced way. Does it adhere to all regulations such as GDPR? Has it been suitably scrubbed of any personal information? If a data vendor can’t answer this, then investors cannot use the dataset at all.
Data buyers want support and explanation
Data buyers want to understand what a dataset is. They want to know what all the fields are and how the data has been recorded. What is the lag like when releasing the data? Has the data been fetched from an approved licensed source? It isn’t possible to look at data and discern all of this. The data vendor needs to be ready to explain the dataset to the buyer. Having research papers already done on the dataset, can also help data buyers understand use cases for the data (and yes Cuemacro can help data vendors with white papers, contact me if you’re interested). If a data vendor makes no attempt to help a client understand the data, it will take a lot more time for the client to evaluate that dataset. In practice, they might not even try to take a trial, if it takes too long.
Conclusion
There is no one size fits all for data. However, there are broad criteria which can be used by investors for datasets and they go far beyond what we’ve discussed here (see the forthcoming book for much more detail!) If a dataset seems to tick all the boxes from such a checklist, then an investor can start looking at the dataset in more detail (eg. backtesting to assess the presence of alpha).