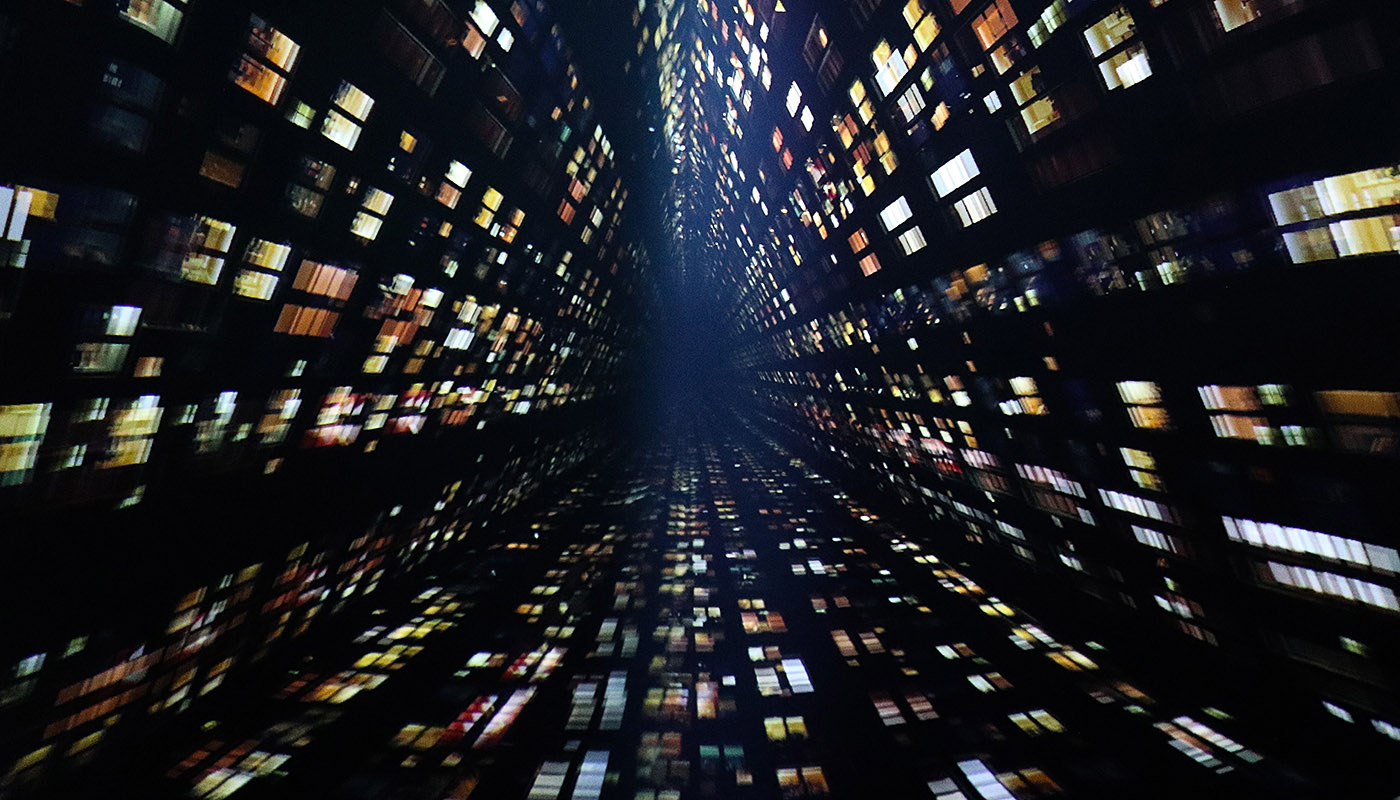
The weather is one of those universal neutral conversation starters, at least it in the United Kingdom (and well, let’s just steer clear of that conversation topic called Brexit for a few minutes). Hot weather isn’t always desirable. I was recently in Geneva, and had been expecting very cold days, bracing myself for a torrent of snow. Yet, in practice, I was pleasantly surprised that it was hotter than I had expected. I had been told, that this unseasonably hot weather had been pretty bad news for skiing. Your viewpoint about the weather depends on what your doing. If you’re walking around between work meetings, avoiding snow is a plus. If you’re skiing, snow is kind of desirable!
Data is like that. Everyone had different needs for their data. Obviously, there are some properties of data which are desirable for everyone, like accuracy and making sure that the data has been properly sourced. This of course includes ensuring that the data adheres to all laws, in particular around PII (personal identifiable information). However, aside from that financial users have many differing criteria for their data. High frequency traders need (as the name suggests!) high frequency data for the markets they trade, and potentially for markets they don’t.
Data scientists working for discretionary asset managers will be investigating firms in a lot of depth. Hence they will be looking for many datasets to enable them to drill down into a small number of assets. By contrast, quant funds will be looking for datasets that work across many assets, to enable analysis at scale.. They can take advantage of being able to automate analysis and also the ability to execute very complicated portfolios electronically. Risk managers need market data to input into their risk models. Typically it doesn’t need to be delivered in realtime, but still needs to be accurate!
The role of a data strategist in a fund is to help work with internal stakeholders, such as data scientists, portfolio managers and traders to identify the types of datasets they would like. They will then go out to data vendors to try to find the most appropriate datasets. Given that internal needs can vary considerably between groups, the role of a data strategist is pretty difficult. It requires many different skillsets including:
- technical – understanding how the data will be used
- commercial – negotiating with vendors
- legal & compliance – working with legal to do due diligence on datasets
- management – to coordinate many different stakeholders both internally and externally
Data isn’t a one size fits all. Every user will have different requirements in terms of data. Just because one dataset is super useful for one team, doesn’t mean that it will be for another. Data vendors need to be aware of this when pitching their data, to identify potential users and understand use cases. Data strategists in funds are a key part of this process to funnel the right data to the right teams.