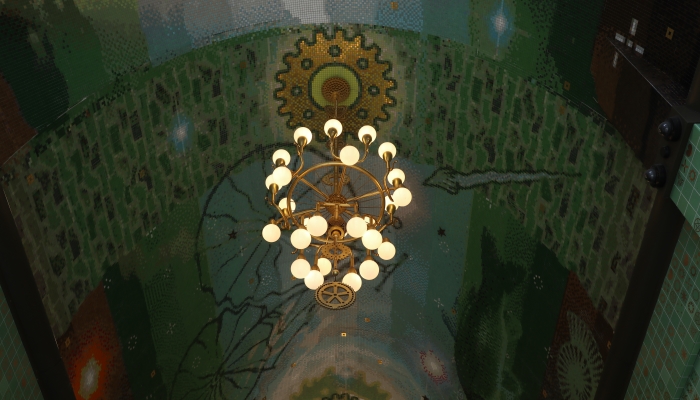
I’ve got a car, despite the fact that I rarely drive it. There’s that nagging feeling, that I should keep my car, just in case I need it. On those rare occasions I do drive it into London, I end up moaning about all the traffic, and wish I’d taken the Tube. Not only that, but it’s more polluting than public transport, because it’s a petrol car. Furthermore, I’ve got to find a parking space, which is both rare and costly. Whilst having a car might give me more “freedom” to travel, all these other factors actually seem to counter that freedom. If there was no traffic and I had an electric car, maybe driving might make more sense.
Ultimately, my point is that having a car in isolation, might allow you to drive, but there are many other factors which are necessary to make that a nice experience. In a sense, this is like the use of alternative datasets within finance. There are many conditions which are necessary for alternative data to be effective within the investment process. There are lots of folks who bemoan alternative data, because datasets don’t always have a “magic” trading signal on the surface. Yes, there are many alternative datasets, which don’t have much of a signal, and yet there’s a significant cost to obtaining these datasets. Just because data is rare and unusual doesn’t necessarily mean there’s a signal. However, this kind of misses the point. On many occasions, to get signals from alternative datasets (or indeed more common datasets), the trick isn’t just finding that special dataset which is the “secret sauce” in isolation.
The trick is joining together different useful datasets to create an enhanced signal, to help crosscheck each other. Alex Denev and I are currently coauthoring The Book of Alternative Data, which will be published by Wiley in 2020 (Amazon pre-order here). We’ve written a substantial part of the book already. We introduce the topic of alternative data, and go through the various challenges associated with its usage. Many of these challenges might be common to “ordinary” data, such as dealing with missing data points. Later in the book, we go through many different alternative data use cases for investors, ranging from automotive supply chain data to news data to satellite data to web traffic data etc.
In many examples, we look at specific datasets in isolation to understand how they can be used to understand markets. In some cases, we look at using multiple datasets together, to see whether they complement each other. For example, we combined car count data for the car parks of European retailers, together with news sentiment on those same companies. Whilst, each dataset in isolation has a good R^2 for helping to estimate earnings per share, we find that combining them increases the R^2. This seems intuitive given they are ultimately different measures of a company’s performance. I’m sure that adding other datasets into the mix would likely help to increase the performance of the model.
Hence, the whole point with alternative data isn’t only what datasets you are using, but how you are using them together and which make sense to combine. That is where the real alpha is, having an understanding of which datasets you can combine together. What might seem like a weak signal in isolation, may actually be a nice (uncorrelated) addition to your existing framework. It obviously helps to know which key performance indicators you are hoping to measure (eg. is it earnings per share, PMIs etc.), and how those datasets can help you. This requires a lot of domain knowledge and for data scientists to work together with portfolio managers and traders. If you’re interested in alternative data and how it can help your investment process, drop Cuemacro a message! We can also come to your office to do an alternative data bootcamp if that’s of interest.