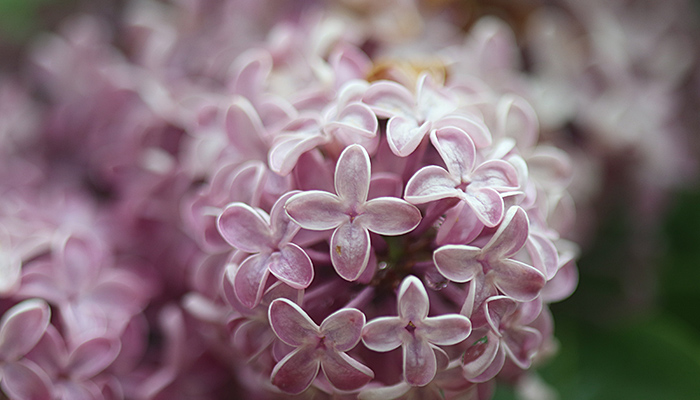
I know how to find a decent burger. I’ll scour the web, I’ll walk around, I’ll ask my friends. If there’s a good burger, I’ll be sure to find it. When it comes to cooking it, well, I’m probably not quite as confident. Sure, I can make a burger. Will it be the best burger, the finest you’ve ever eaten? I somehow doubt it. Everybody has their own skill set. Finding a burger and eating it, as a skill (ok, perhaps, it isn’t really a skill) is somewhat different to actually cooking the burger. The notion that everyone can’t do everything as well, is of course pretty obvious.
When it comes to data, the same is true, that we can’t do everything. I recently had lunch with Rob Passarella (and he also happens to know where the best cheesecake is in New York City). He’s been in the alternative data industry for a number of years, with exposure to many different datasets including machine readable news, and he knows the area of alternative data extremely well. We’ve all heard of the analogy of data as being the new oil, and it’s been cited in many places including The Economist. The idea being that we can extract value from data, in the same way that those sitting on oil have done. During our discussion Rob tried to extend this notion of data being oil, into an analogy of the whole data industry (and thanks Rob for inspiring this article!). He later posted the idea as a tweet, which I’ve copied below.
If Data is the new Oil – we should think about the industry as: Upstream – Exploration & Production, Mid-Stream – Transport & Storage, & Down Stream – Refining & the Customer … this way we know where the players fit
— Rob Passarella (@robpas) May 1, 2019
The idea is obviously, that the whole data pipeline is split in the same way that the oil industry is. Each part of the pipeline needs different skills. Data is in the “ground”. It might be “exhaust data” generated by other processes. It be data generated by corporates. It could be data generated by individuals generated by their online activity. It could be data generated as a result of trading on exchanges. Typically, the “ground” will be data lakes which are near the source of data, for example in the actual corporate firm where it was generated. Often this data will be in its “crude” form, unstructured and difficult to decipher.
The upstream part of the pipeline, will seek to find these raw datasets. These data firms will often seek to collect datasets from a multitude of different sources. The next stage will be to store this data, the mid-stream stage. The downstream part of the pipeline is where this raw data has already been collected. It is now ready to be refined, which involves structuring the “crude” into a more usable form. Structuring involves making each of the data sources into a more common and usage form. It can involve mixing the various datasets together, adding metadata. Finally, the refined data is ready to be delivered to the customer. The customer, such as a hedge fund firm, will be ready to ingest the “refined” data and extract specific insights from it to generate trade ideas.
Just as with my burger analogy, each part of the this pipeline, clearly requires different skills. The upstream part needs a lot of creativity to seek out specific datasets and the ability to build relationships with the ultimate data owner. The midstream part is heavily data engineering intensive. It requires the ability to store and manage massive datasets, and in particular the construction of data lakes for unstructured datasets. This needs data engineering. The downstream sectors requires a lot of expertise around data science and also some domain knowledge concerning the final usage. Structuring requires an ability to properly do entity matching and other time consuming tasks.
The customer will clearly be the one who leverages an even greater part of their domain knowledge. Over time, it is likely that firms that were originally purely “explorers”, will seek to go further downstream. Firms that generate the data may move to become more involved in exploration and in the mid-stream stage. From the other side, customers can also seek to move further upstream, seeking to get access to the raw data itself, at the exploration stage. Indeed, for more freely available data, such as web scraped data, there are less barriers for funds to get involved in collecting the raw data. However, the barrier is in the ability to structure this data at scale.
If you’re interested in alternative data and how it can be used to help your business, in particular if you are in a financial firm, please drop me message to see how Cuemacro can help. Alex Denev and I are writing The Book of Alternative Data and Henry Sorsky is helping us with some of analysis. The book is due out in 2020. We’ll be giving a more detailed take on some of the ideas discussed here and in my other Cuemacro posts, in the book.