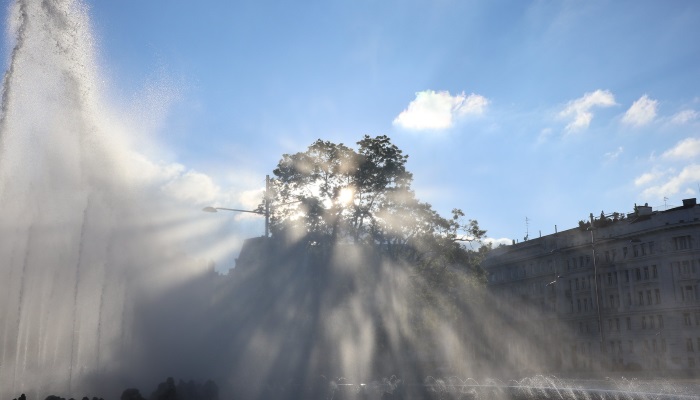
Vienna is one of those places which has somehow largely eluded my travels. The last time I visited it was 25 years ago. However, it has very much stuck in my memory. History is one of those things which you can never escape from in Vienna. The echos of musical history are everywhere, whether it is Mozart or Beethoven, or the Viennese waltz. There are also the very well known difficult periods of war which the city went through in the twentieth century. When I visited it recently, whilst the city had evidently changed, I was happy that many of my memories of the place were intact. I also indulged in perhaps a bit too much of the heaviest types of Viennese food (Schnitzel, cake and Tafelspitz). The main reason I had come to Vienna, however, was for the annual QuantMinds conference. I’ve been coming to the QuantMinds conferences for a number of years. What has been noticeable is how the conference has changed. During my first few years, the focus was very much on the traditional areas of quantitative finance, mainly option pricing. Of course, option pricing is still a key area for the conference, but the agenda in more recent years has often been punctuated by machine learning and other newer areas such as alternative data.
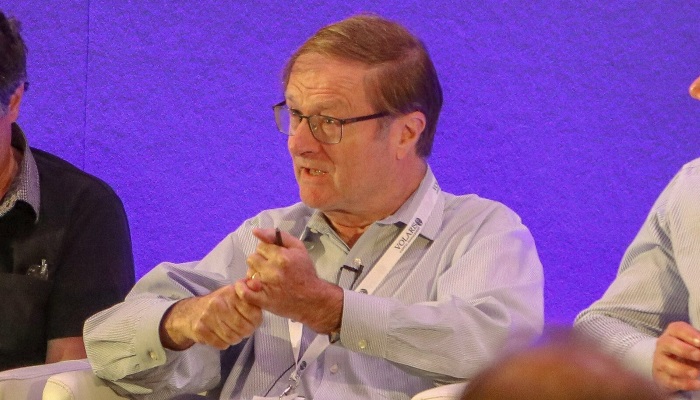
The headline panel of the event had some of the best known quants in the industry, Damiano Brigo (Imperial College), John Hull (University of Toronot), Peter Carr (NYU Tandon) and Vladimir Piterbarg (NatWest Markets). The major topic was how new trends were impacting quant finance. On the subject of machine learning there was still largely scepticism. Peter Carr noted that it wasn’t new, citing papers which were 20 years old, which were using ideas like neural networks to do option pricing. The difficulties of interpretability were also flagged by Brigo.
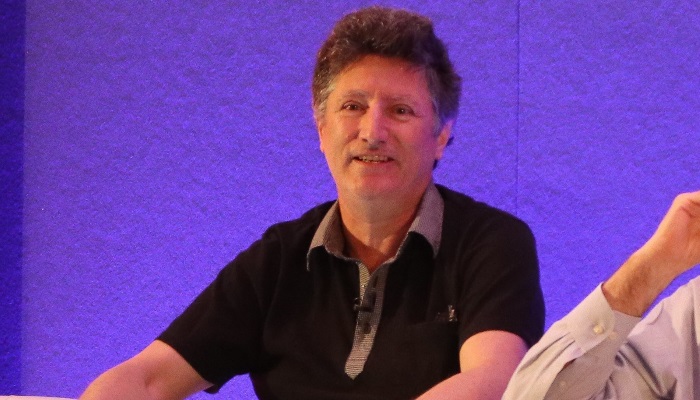
Piterbarg noted how advances in machine learning in other areas like Google Translate and image recognition, were not matched by equally impressive advances in finance. Whilst, there might be applicability for high frequency datasets, it was not as easy for lower frequency data, such as daily. However, John Hull very much disagreed, flagging how machine learning was being used to speed up numerical solving. He advised people to learn as much as possible on machine learning, and he said it would have a big impact on finance. He has spent the past 12-18 month learning about machine learning and he is now writing a book on the subject. He noted that in finance in practice, we already use many black box models and there were new techniques being researched to look at the issue of interpretability.
The area of quantum computing also cropped up in a talk by Davide Venturelli. He stressed that we are still very far away from a quantum computer which can be used to break RSA encryption. Whereas bits in computing can 0 or 1, qubits, their quantum equivalent can be both 0 and 1 at the same time. They are easy to create, he noted, but they are very difficult to control. Hence, the probability of errors are very high, and hence error correction needs to be employed to use them. One interesting point he noted was that quantum computing was not purely about speed. A quantum computer also used a lot less energy.
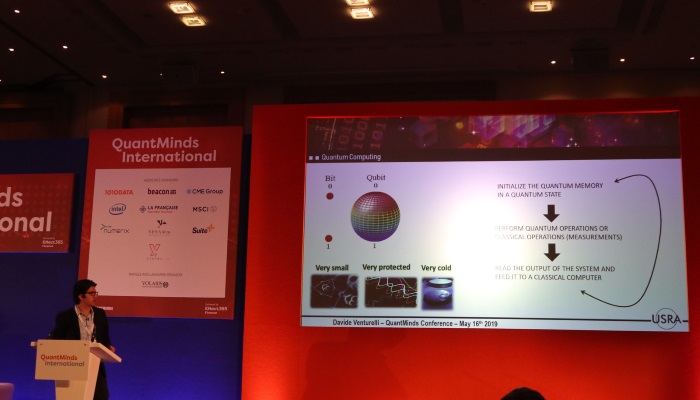
There were a number of different talks about market microstructrure and related topics like transaction cost analysis from academics and practitioners such as Mathieu Rosenbaum who spoke on “Recent quantitative advances in market making regulation”. Rama Cont (Oxford) also presented on market impact, rethinking the notion that it was proportional to the square root function. Zoltan Eisler (CFM) presented research on how crowding and market impact. His point was that market impact could not be measured in isolation for an individual trader. In practice, we should measure co-impact from multiple traders doing the same strategy. Stefano Pasquali (BlackRock) discussed how his firm was trying to measure liquidity risk, which included trying to understand how to model transaction costs. He presented some novel ideas for estimating transaction costs in less liquid markets like credit, including using NLP, to interpret trader’s explanations why the “model” price was different from where they transacted. He noted that whilst a neutral model could improve the performance of forecasting transaction costs, it was also not interpretable to explain to traders.
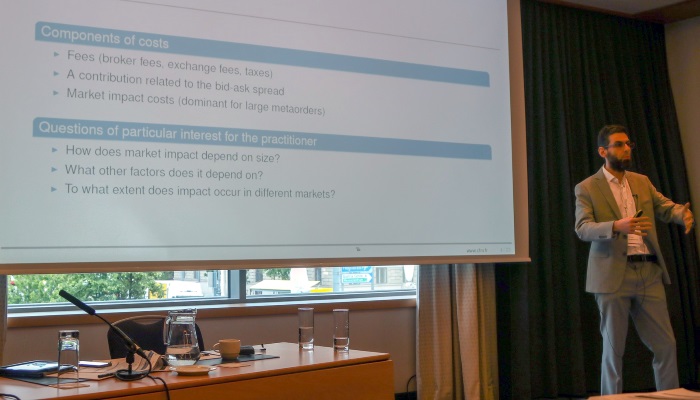
Machine learning featured (excuse the pun!) in a number of different talks more broadly. Marcos Lopez de Prado’s talk was a general overview of the topic. He noted how machine learning could be used to model non-linear relationships and could handle unstructured data. However, there was a need to be careful when dealing with time series. He cited several examples, including portfolio construction. He also noted that it could be used for parts of the investment process, for example, in price prediction, to size bets (in combination with another model for the trade direction). Patrik Karlsson and Hanna Hultin (SEB) talked about how it was possible to use reinforcement learning to help simulate the execution process to develop algos. Also on the trading front, Mark Higgins (Beacon) discussed the difficulty with hedging the right to use natural gas storage facilities. There were many questions which traders had to ask, such as where to hedge the natural gas risk (at a liquid hub or at the storage facility, but this could impact basis risk). There were also many other factors such as seasonality. He showed how it was possible to use a neural network which could allow better for hedging than Black-Scholes. Richard Turner (Mesirow Financial) also presented ways of doing portfolio optimisation for FX strategies looking at expected shortfall and also introducing how machine learning might be applied in this area.
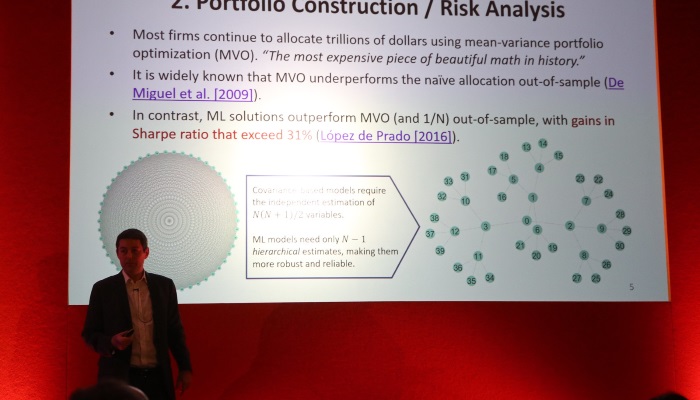
We often think of machine learning as a way of developing a non-linear model to describe relationships between variables. However, there were a number of talks at QuantMinds which instead tried to use machine learning to help evaluate existing models. Katia Babbar (AI Wealth Technologies) discussed how to use deep learning to price exotic options. The objective in using a deep neural network was to make the pricing of options more efficient, ie. using the same pricing models, but having a more efficient numerical solution. Blanka Horvath (Kings College), Mehdi Tomas (Ecole Polytechnique) and Aitor Muguruza (Imperial College) presented a way to calibrate rough volatility models in a few miliseconds for the whole implied volatility surface. The idea was to do the learning step offline, and the coefficients of the learned model could be used later to do (fast) calibration when needed. Matthew Dixon (IIT) presented a paper on how to use Gaussian processes to do CVA computation. On the subject of interest rates, Marcos Carreira (Ecole Polytechnique) discussed various schemes for interest rate interpolation. When it came to learning from your dataset, he noted that it was important to know which regime you were in.
Taking a totally different angle on the use of machine learning, Daniel Mayenberger (Barclays) talked about its use in detecting credit card fraud. As many other speakers had noted throughout the conference, he said that many of the techniques in machine learning weren’t actually that new. When it came to feature engineering an early step could be to visualise the data to get initial ideas. All models were based on assumptions, and potentially sensitivity tests could be used to understand how they behaved.
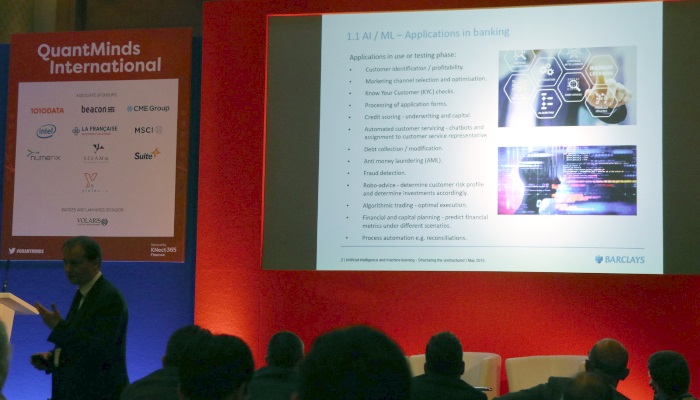
Machine learning needs data! Hence, it’s not surprising that in recent years the area of alternative data has become a key area in finance. I introduced the topic of alternative data in my talk. The main objective was to discuss how alternative data could be valued and to give some use cases, which included using CLS FX flow data to trade FX and the use of Bloomberg News articles to generate FX signals. Matt Napoli (1010 Data) talked also talked about alternative data, and in particular at the datasets his firm distributed, which revolved mostly around credit card transaction data. He explained the difficulties in structuring this data, with appropriate tagging and how his firm had invested a lot of time in doing this.
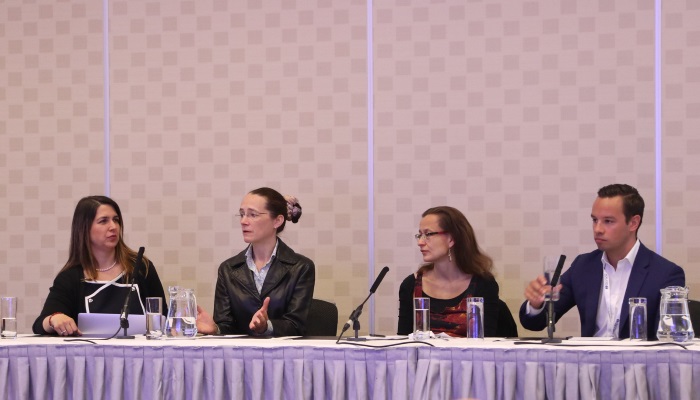
The quant industry is diverse in some ways, but certainly not in others, in particular in terms of gender balance. A panel made up of Oliver Cooke (Selby Jennings), Katia Babbar (AI Wealth Technologies), Birgit Radloff (Vienna University) and Jessica James (Commerzbank) discussed the topic of diversity. Cooke noted that only 8% of quants are women. It was noted that whilst diversity was the right thing to do, there was also a business case for it. Whilst progress was being made, it wasn’t quick enough. Flexible working was also discussed.
What will be at QuantMinds next year? It’s obviously difficult to tell, but I think it’s a reasonable forecast that there will be more about machine learning and data science in general. I’ll very much hope that I’ll be able to see what will be discussed at QuantMinds in 2020.